Artificial Intelligence (AI) has emerged as a transformative technology with immense potential across various industries. From healthcare to finance and beyond, organizations are embracing AI solutions to enhance efficiency, streamline processes, and drive innovation. However, with the plethora of options available in the AI landscape, finding the perfect AI solution for your specific needs can be a daunting task. In this article, we will navigate the AI landscape and provide insights on how to choose the best AI solution for your requirements.
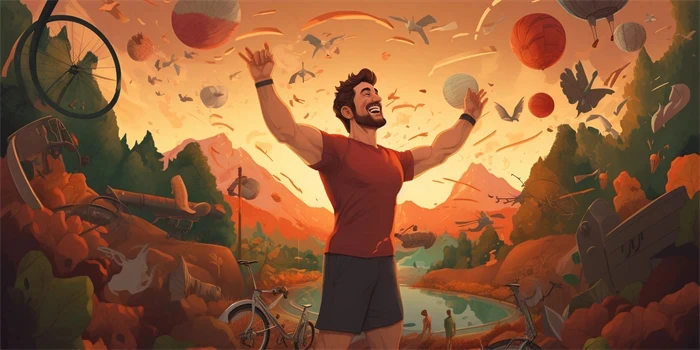
1. Define Your Objectives
Before embarking on your search for an AI solution, it is crucial to clearly define your objectives. Identify the specific problems you aim to solve, the outcomes you expect, and the potential areas where AI can add value to your business.
2. Assess Data Availability and Quality
AI algorithms require vast amounts of high-quality data to train and generate accurate insights. Evaluate the availability and quality of your data to determine if it meets the requirements of the AI solution you are considering. If data gaps exist, consider strategies to collect and preprocess the data.
3. Evaluate Scalability and Integration
Consider the scalability and integration capabilities of the AI solution. Will it be able to handle increasing data volumes and evolving business needs? Does it seamlessly integrate with your existing systems and infrastructure? These factors are crucial for ensuring a smooth implementation and long-term success.
4. Analyze Training and Deployment Processes
Look into the training and deployment processes associated with the AI solution. How long does it take to train the model? Does the deployment process align with your timeline and resource availability? Understanding these processes helps manage expectations and aids in planning.
5. Consider Ethical and Legal Implications
AI solutions can have ethical and legal implications, especially when dealing with sensitive data. Ensure that the solution adheres to relevant regulations and industry standards. Consider the potential biases and fairness of the AI system, as well as privacy and security measures implemented.
6. Evaluate Performance and Accuracy
Assess the performance and accuracy of the AI solution by evaluating its track record and conducting proof-of-concept tests. Consider factors such as precision, recall, and F1 score, depending on the specific AI application. Look for case studies and references to ensure the solution delivers on its promises.
7. Understand Customization and Support
Consider the level of customization and support provided by the AI solution. Can it be tailored to meet your specific needs? Is there a dedicated support team available to address any issues or concerns? Understanding these aspects ensures a smooth implementation and ongoing support.
8. Analyze Cost and ROI
Take into account the cost of the AI solution and the potential return on investment (ROI). Consider the upfront costs, ongoing fees, and the potential savings or revenue generation the solution can offer. Conduct a thorough cost-benefit analysis to make an informed decision.
FAQs:
Q1: What are some popular AI tools for data analysis?
A1: Some popular AI tools for data analysis include IBM Watson, Google Cloud AI, and Microsoft Azure Machine Learning. These tools offer a range of functionalities, from data preprocessing to advanced analytics.
Q2: How long does it typically take to train an AI model?
A2: The time required to train an AI model varies depending on factors such as dataset size, complexity of the problem, and computational resources. It can range from a few hours to several weeks.
Q3: What are some ethical considerations when using AI?
A3: Ethical considerations when using AI include ensuring fairness and avoiding biases, protecting privacy and security, and complying with regulations and legal requirements.
References:
1. Smith, J. (2019). The AI-powered Enterprise: Unlocking the Potential of Artificial Intelligence. HarperBusiness.
2. McKinsey & Company. (2021). Artificial Intelligence. Retrieved from https://www.mckinsey.com/ai
3. Gartner. (2021). Gartner AI Technology Hype Cycle. Retrieved from https://www.gartner.com/en/documents/3997171/ai-technology-hype-cycle-2021