Artificial Intelligence (AI) has transformed various industries, promising to make systems smarter and more efficient. However, there is a growing concern about the bias that can be embedded in AI algorithms and the ethical challenges it presents. In this article, we will explore the concept of AI bias, its implications, and strategies to address these ethical challenges.
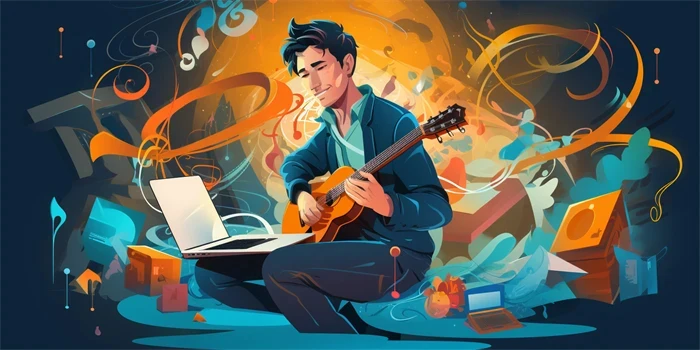
Defining AI Bias
AI bias refers to the systematic and unfair favoritism or prejudice shown by AI systems towards or against certain individuals, groups, or characteristics. It can arise from biased training data, flawed algorithms, or inadequate testing procedures. Understanding different aspects of AI bias is crucial to mitigate its potential adverse consequences.
The Implications of AI Bias
1. Reinforcing societal biases:
AI systems learn from data, which may already contain societal biases. When biased data is used to train AI algorithms, it can perpetuate discriminatory practices or reinforce existing inequalities in society.
2. Discriminatory decision-making:
AI systems can make decisions that are unfair or discriminatory, such as in hiring practices or loan approvals. If not handled carefully, AI bias can exacerbate societal discrimination, leading to ethical dilemmas.
3. Lack of diversity and inclusivity:
Biased AI can prevent diverse voices and perspectives from being properly represented. If AI systems are not designed to be inclusive, they may end up serving only a specific portion of the population, excluding others.
Causes of AI Bias
1. Biased training data:
AI algorithms learn patterns from training data. If the training data is biased, the AI system will inevitably reproduce those biases, leading to biased outcomes.
2. Unintentional human biases:
Developers and data scientists may unknowingly introduce their own biases into AI systems during the design and development process. These unconscious biases can then become embedded in the algorithm itself.
3. Inadequate testing and validation:
Failure to rigorously test and validate AI systems can allow biased algorithms to go unnoticed. Ethical challenges arise when biased AI is deployed in real-world applications, impacting individuals and communities.
Addressing AI Bias
1. Diverse and representative training data:
Ensuring that training data is diverse and representative of different groups and characteristics can help minimize bias. It is crucial to address underrepresented communities and include their perspectives in the data collection process.
2. Transparent AI algorithms:
Developers should strive for transparency in AI algorithms. By making the algorithms open and accessible, it becomes easier to identify and address biases. Transparency also fosters trust and accountability in AI systems.
3. Continuous monitoring and evaluation:
Regular monitoring and evaluation of AI systems can help identify and rectify any biases that may emerge over time. This ongoing process ensures that biases are addressed promptly, reducing potential harm.
4. Ethical guidelines and standards:
The development and deployment of AI systems should adhere to ethical guidelines and standards. These guidelines can help guide developers in creating unbiased AI and ensuring responsible and fair practices.
5. Human oversight and intervention:
While AI systems can automate many processes, human involvement is crucial in mitigating bias. Human oversight and intervention can help identify and correct biases that AI may overlook.
Frequently Asked Questions
Q: Can AI completely eliminate bias?
A: While AI can help mitigate bias, complete elimination is challenging as biases sometimes stem from societal issues. However, by adopting ethical practices and strategies, we can minimize and address bias effectively.
Q: Are there any legal consequences for biased AI?
A: Depending on the jurisdiction, biased AI can lead to legal consequences. Discriminatory practices and violations of privacy and equal opportunity laws can result in legal action against organizations using biased AI systems.
Q: Can AI bias be unintentional?
A: Yes, AI bias can be unintentional. Developers and data scientists may have unconscious biases that inadvertently get embedded into the AI algorithms during the design and development process.
References
1. Caliskan, A., Bryson, J. J., & Narayanan, A. (2017). Semantics derived automatically from language corpora contain human-like biases. Science, 356(6334), 183-186.
2. Narayanan, A. (2018). AI2: AI for social good. AI Magazine, 39(2), 9-20.
3. Zou, J. Y., Schiebinger, L., AI100 Standing Committee and Stanford University, Stanford, CA. (2018). AI as a feminist issue: past commitments and future directions AI Matters, 4(2), 12-21.