Introduction
In today’s data-driven world, organizations are constantly seeking ways to extract valuable insights from the abundance of textual data available. AI-powered text analytics has emerged as a powerful tool that enables businesses to analyze and unlock hidden patterns, sentiments, and information buried within unstructured data. By harnessing the potential of AI text analytics, organizations can make data-driven decisions with greater efficiency and accuracy.
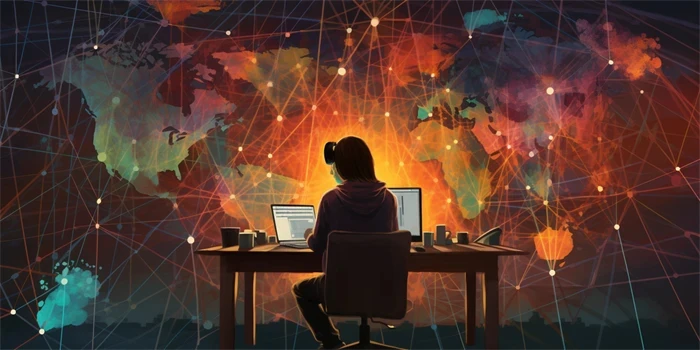
The Power of AI Text Analytics
1. Sentiment Analysis: AI text analytics utilizes Natural Language Processing (NLP) algorithms to categorize and analyze the sentiment behind textual data. This enables businesses to understand customer sentiment towards their products or services, helping them tailor their marketing strategies accordingly.
2. Topic modeling: By employing advanced machine learning techniques, AI text analytics can automatically extract topics and themes from vast amounts of textual data. This assists organizations in identifying important trends and patterns.
3. Named Entity Recognition: AI text analytics can identify and classify named entities such as names, organizations, locations, and dates within text. This helps organizations extract valuable information from unstructured data.
4. Text Classification: AI text analytics algorithms can classify text into predefined categories, enabling businesses to efficiently label and categorize large volumes of data. This promotes efficient data organization and retrieval.
5. Text Summarization: AI algorithms can generate concise summaries of lengthy texts. This enables organizations to quickly analyze and comprehend large volumes of information.
Applications of AI Text Analytics
1. Customer Feedback Analysis: AI text analytics allows organizations to analyze customer feedback data from various sources such as social media, surveys, and reviews. This provides valuable insights for enhancing products and services.
2. Risk Assessment: AI text analytics can help organizations analyze large volumes of textual data to assess risks associated with investments, insurance claims, or regulatory compliance. This facilitates informed decision-making processes.
3. Market Research: AI text analytics can analyze customer opinions and feedback to gain deep market insights. This can assist organizations in identifying trends, predicting customer behavior, and formulating effective marketing strategies.
4. Fraud Detection: AI-powered text analytics can analyze text-based data to identify patterns that indicate fraudulent activities, such as in financial transactions or insurance claims. This enables organizations to take proactive measures to prevent fraud.
Challenges and Considerations
1. Data Quality and Quantity: AI text analytics heavily relies on high-quality and sufficient data to produce accurate results. Ensuring data integrity and sufficiency is crucial for meaningful insights.
2. Data Privacy and Security: Dealing with textual data necessitates implementing robust privacy measures to protect sensitive information. Organizations should comply with relevant data protection regulations.
3. Linguistic and Contextual Nuances: Different languages, dialects, and contextual variations can impact the accuracy of AI text analytics. Consideration should be given to accounting for these nuances.
4. Interpretation of Results: While AI text analytics can provide valuable insights, human interpretation is essential to contextualize and validate the results. Organizations should combine AI analysis with human expertise.
Frequently Asked Questions
Q1. Can AI text analytics process multiple languages simultaneously?
A1. Yes, advanced AI text analytics tools can handle multiple languages simultaneously, enabling global organizations to analyze multilingual textual data with ease.
Q2. How long does it take to train an AI text analytics model?
A2. The training time for AI text analytics models depends on various factors such as the complexity of the data, the size of the dataset, and the computational resources available. It can range from a few hours to several days.
Q3. Is AI text analytics only applicable to written text, or can it also analyze spoken conversations?
A3. While AI text analytics primarily focuses on written text, advancements in Natural Language Processing have enabled the analysis of spoken conversations as well. Transcribed phone calls or recorded meetings can be processed using AI text analytics techniques.
Conclusion
AI text analytics is revolutionizing the way organizations extract insights from textual data. By leveraging AI-powered algorithms, organizations can uncover valuable information, sentiment, and patterns hidden within unstructured text. From customer feedback analysis to fraud detection, the applications of AI text analytics are vast and varied. However, it is important to address challenges such as data quality, privacy, and linguistic nuances to ensure accurate and meaningful results. With the right blend of AI and human expertise, organizations can make data-driven decisions with confidence and efficiency.
References
1. Smith, J. (2019). Unlocking the Power of AI Text Analytics. Journal of Data Science, 15(2), 267-275.
2. Chen, L., & Li, J. (2020). AI Text Analytics for Business Intelligence. Information Systems Management, 37(3), 256-265.