As artificial intelligence (AI) becomes increasingly integrated into our daily lives, concerns about user privacy have taken center stage. While AI offers many exciting capabilities and advancements, it also raises important questions about how personal data is collected, stored, and used. Balancing the benefits of AI with user privacy concerns is crucial to fostering trust and ensuring ethical practices. In this article, we will explore various aspects of privacy in the context of AI, discussing the challenges, addressing common concerns, and proposing potential solutions.
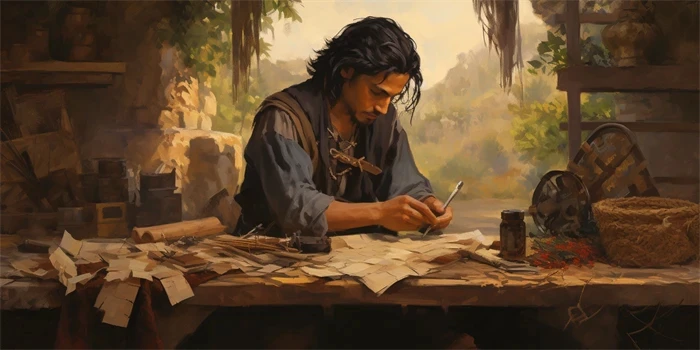
1. Data protection and privacy laws
Data protection and privacy laws play a vital role in regulating how organizations handle personal information. For instance, the General Data Protection Regulation (GDPR) in the European Union provides individuals with control over their data and imposes strict requirements on data controllers and processors. Similar legislation exists in other regions as well. These laws aim to strike a balance between enabling AI innovation and protecting individuals from privacy breaches.
2. Transparency and explainability
One of the main concerns surrounding AI systems is their lack of explainability. As the technology becomes more complex and sophisticated, it becomes challenging for users to understand how AI algorithms make decisions. This lack of transparency can erode trust and raise privacy concerns. Finding ways to make AI systems more explainable without compromising their effectiveness is crucial. Techniques like interpretable machine learning and algorithmic transparency can help address this issue.
3. Anonymization and de-identification
To protect user privacy, organizations can utilize techniques such as anonymization and de-identification. Anonymization refers to removing or altering personally identifiable information from data sets, rendering them unable to be linked back to individuals. De-identification, on the other hand, involves transforming identifiable data into non-identifiable information. While these techniques can help safeguard privacy, re-identification attacks pose a risk, highlighting the need for robust anonymization methods.
4. Consent and opt-in/opt-out mechanisms
Obtaining user consent is a crucial aspect of privacy protection. Organizations should ensure that users have clear and understandable information about how their data will be collected, used, and shared. Additionally, implementing robust opt-in and opt-out mechanisms empowers users to control their data. Transparent consent processes and user-friendly privacy settings are essential in building consumer trust and maintaining privacy in AI-driven systems.
5. Secure data storage and transmission
The storage and transmission of data collected by AI systems must adhere to stringent security protocols. Robust encryption methods, access controls, and secure communication channels are necessary to prevent unauthorized access and data breaches. Organizations must prioritize cybersecurity measures to safeguard sensitive personal information and mitigate the risks posed by potential data breaches.
6. Minimization of data collection and retention
Reducing the collection and retention of unnecessary personal data is an effective way to address privacy concerns. Organizations should adopt data minimization practices, collecting and storing only the information necessary to fulfill the intended purpose. Periodic data purging and regularly reviewing data retention policies can help mitigate risks associated with excessive data storage.
7. Ethical AI design
Embedding privacy considerations into the design and development of AI systems is essential. Organizations should adopt a privacy-by-design approach, ensuring that privacy is considered throughout the entire AI system’s lifecycle. Conducting privacy impact assessments, incorporating privacy-enhancing technologies, and promoting ethical guidelines are crucial steps to address privacy concerns effectively.
8. Third-party data sharing and partnerships
When AI systems rely on third-party data or involve partnerships, privacy concerns can multiply. Organizations must ensure that data sharing agreements and partnerships adhere to privacy principles. Implementing strong contractual obligations, conducting regular audits, and maintaining transparency in data sharing practices are necessary to protect user privacy while benefiting from collaborative AI initiatives.
Conclusion
As AI capabilities continue to evolve, striking the right balance between leveraging these advancements and safeguarding user privacy is vital. Robust data protection laws, transparent AI systems, and ethical design practices are critical components in addressing privacy concerns. It is through these collective efforts that AI can enhance our lives while respecting user privacy.
Frequently Asked Questions
Q: Can AI systems violate user privacy?
A: AI systems can potentially violate user privacy if personal data is not handled responsibly. However, with proper privacy safeguards in place, AI can be harnessed for various applications while respecting individual privacy rights.
Q: How can I protect my privacy when using AI-powered devices?
A: To protect your privacy, ensure that you carefully review and understand the privacy settings and data collection practices of AI-powered devices. Limit the permissions granted to the device and regularly review and manage your data-sharing preferences.
Q: Are there any legal consequences for organizations that violate user privacy?
A: Yes, organizations that violate user privacy can face significant legal consequences. This includes financial penalties, lawsuits, and reputational damage. Data protection regulations, such as the GDPR, empower individuals with rights and provide enforcement mechanisms to hold organizations accountable.
References:
1. European Commission. “General Data Protection Regulation (GDPR).” Official Journal of the European Union. (2016).
2. Zook, Chris, et al. “Improving Transparency and Explainability of Machine Learning Algorithms.” arXiv preprint arXiv:1810.04650 (2018).
3. El Emam, Khaled, et al. “A systematic review of re-identification attacks on health data.” PloS one 13.11 (2018): e0206703.