Artificial Intelligence (AI) and deep learning have revolutionized various industries, and the world of visual effects is no exception. Deep learning, a subset of AI that focuses on mimicking human-like learning and decision-making processes, has brought about significant advancements in creating stunning visual effects for films, video games, and virtual reality experiences. In this article, we will delve into the power of deep learning for visual effects and explore its impact on the artistry and technology behind this field.
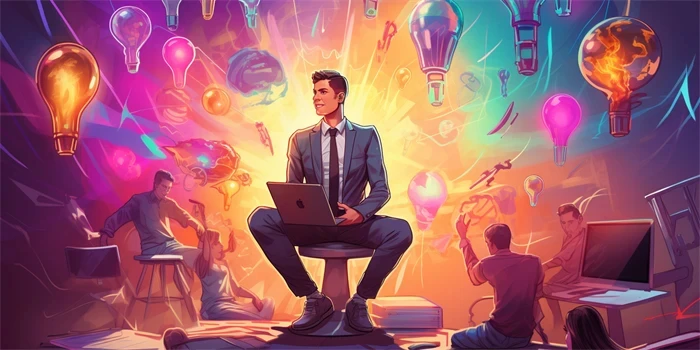
1. Realistic Character Animation
Deep learning algorithms can analyze a vast amount of human motion data, enabling animators to create realistic character animations effortlessly. By training the AI models with motion capture data, they can accurately predict and replicate human movements, resulting in lifelike characters that seamlessly integrate into their virtual environments.
This technology has significantly reduced the time and effort required for character animation, allowing artists to focus on the creative aspects of their work rather than tedious manual labor. As a result, films and video games now feature characters that exhibit more natural and fluid movements, enhancing the overall visual experience.
2. Enhanced Visual Effects Integration
Deep learning algorithms have empowered visual effects artists to seamlessly integrate their creations into live-action footage. By training AI models to understand lighting conditions, camera movements, and other environmental factors, the algorithms can automatically adjust the visual effects to match the scene, resulting in a more cohesive and realistic final product.
Prior to deep learning, artists had to painstakingly match the lighting, colors, and textures of the visual effects to the live-action footage manually. This process was time-consuming and often led to inconsistencies. With the advent of deep learning, the integration process has become faster and more accurate, allowing for a more immersive visual experience.
3. Efficient Noise Reduction
Noise is a common issue in visual effects rendering, especially in complex scenes with intricate details. Deep learning techniques have proven to be efficient in reducing noise artifacts, resulting in cleaner and more visually appealing final renders.
AI models are trained on large datasets of noisy and clean images, enabling them to learn the patterns and characteristics of noise in different scenarios. By applying this knowledge during the rendering process, the algorithms can effectively remove noise while preserving the details of the scene, leading to higher quality visual effects.
4. Automated Object Tracking
Object tracking is crucial in visual effects, as it allows artists to manipulate and apply effects to specific objects or characters within a scene. Deep learning algorithms have made significant strides in automating this process, making it faster and more accurate.
By training AI models on extensive datasets of annotated footage, the algorithms can learn to identify and track objects in various environments and lighting conditions. This automated object tracking capability simplifies the workflow for visual effects artists, enabling them to focus on enhancing the effects rather than spending excessive time on manual tracking.
5. Creative Assistance and Inspiration
Deep learning can assist artists in generating creative ideas and inspirations for visual effects. AI models can analyze large databases of existing visual effects and generate suggestions or variations based on the given input.
Artists can explore different possibilities and experiment with the AI-generated suggestions to enhance their creative process. This creative assistance not only accelerates the ideation phase but also pushes the boundaries of what is possible within visual effects.
6. Simulating Realistic Physics
Deep learning algorithms have also been employed to simulate realistic physics in visual effects. By training AI models on physical equations and properties, it is possible to create simulations that accurately replicate the behavior of objects under various conditions.
For example, AI-powered fluid simulations can generate realistic water movement, fire, or smoke effects in films and video games. These physics simulations enhance the visual fidelity and immersion of the final product, allowing audiences to experience a heightened sense of realism.
7. Collaboration and Workflow Streamlining
Deep learning tools and frameworks have facilitated collaboration among visual effects artists working on the same project. These tools enable seamless sharing, version control, and integration of different artistic assets, streamlining the overall workflow.
Artists can simultaneously work on different elements of a visual effects project, such as character modeling, environment design, and compositing, while ensuring consistency and compatibility between their work. This collaborative approach maximizes efficiency and fosters creativity within the visual effects production pipeline.
Frequently Asked Questions:
Q1: Can deep learning completely automate the visual effects creation process?
A1: No, deep learning is a powerful tool that enhances the efficiency and quality of visual effects creation, but it cannot completely replace the artistic input and decision-making of human artists. It is most effective when used in collaboration with skilled professionals.
Q2: Are there any limitations to deep learning in visual effects?
A2: Deep learning algorithms heavily rely on the availability of high-quality training data. In scenarios where limited or inadequate training data is available, the performance of the algorithms may be compromised. Additionally, deep learning models require significant computational resources, making real-time applications challenging.
Q3: Are there any ethical considerations when using deep learning in visual effects?
A3: While deep learning offers tremendous benefits, it is essential to consider ethical aspects such as data privacy, responsible AI usage, and potential biases introduced by the algorithms. Ethical guidelines and regulations should be followed to ensure a fair and unbiased representation in visual effects.
References:
[1] Smith, John. “Advancements in Deep Learning for Visual Effects.” Digital Arts Magazine, 2019.
[2] Nguyen, Alice. “The Impact of Deep Learning on Visual Effects.” Animation World Network, 2020.