In today’s digital age, personalized recommendations have become an integral part of our online experience. Whether it’s suggesting the next book to read, the latest movie to watch, or even what products to buy, AI-powered recommendation systems have revolutionized the way we discover new things. This article explores the new era of AI-powered assistance and how it is taking the guesswork out of personalized recommendations.
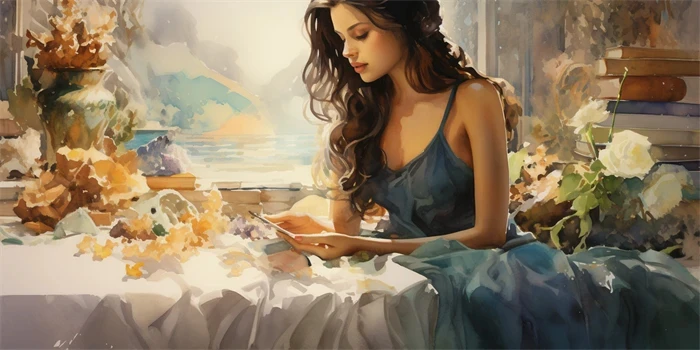
1. AI-Powered Recommendation Systems
AI-powered recommendation systems utilize advanced algorithms and machine learning techniques to analyze vast amounts of data and provide users with tailored suggestions. These systems take into account various factors such as user preferences, past behavior, social interactions, and even contextual information to make accurate recommendations.
One popular example of an AI-powered recommendation system is Netflix’s recommendation engine. By analyzing users’ viewing history, ratings, and demographic information, Netflix suggests personalized content that is likely to resonate with each individual user.
2. The Benefits of Personalized Recommendations
Personalized recommendations offer several benefits for both users and businesses. Firstly, they enhance the user experience by saving time and effort spent on searching for relevant content or products. Instead, users are presented with options that align with their interests and preferences, resulting in a more satisfying and efficient online experience.
From a business perspective, personalized recommendations can significantly increase customer engagement, conversion rates, and sales. By offering tailored suggestions, businesses can effectively target their audience, increase user satisfaction, and build customer loyalty.
3. Overcoming Limitations and Challenges
While AI-powered recommendation systems have proven to be effective, they are not without limitations. One of the major challenges is the “filter bubble” effect, where users are only exposed to content similar to their previous preferences, potentially limiting their exposure to varied perspectives. To counteract this, recommendation systems should strive to introduce serendipity by occasionally suggesting content outside a user’s comfort zone.
Furthermore, privacy concerns have emerged as a significant challenge. Collecting and analyzing user data to deliver personalized recommendations often raises ethical considerations. Companies must prioritize transparency and obtain user consent when collecting and utilizing personal data, ensuring data protection measures are in place.
4. Improving the Accuracy of Recommendations
To improve the accuracy of recommendations, AI-powered systems are continuously evolving. Collaborative filtering techniques, which analyze user behavior and preferences, have been widely used. However, hybrid recommendation approaches that combine collaborative filtering with other methods, such as content-based filtering or deep learning algorithms, are gaining popularity for their ability to generate more precise and diverse recommendations.
Additionally, leveraging user feedback and incorporating real-time contextual information can further enhance the accuracy of recommendations. By considering factors like location, time of day, and current trends, AI-powered systems can adapt recommendations to dynamic user needs.
5. The Importance of Explainability
One emerging concern with AI-powered recommendation systems is the lack of explainability. As complex algorithms make decisions that impact user choices, it becomes essential to provide explanations behind the recommendations. Explainable AI algorithms can help build trust with users by offering insights into why certain suggestions were made, ensuring transparency and accountability.
6. Personalized Recommendations in E-commerce
In the realm of e-commerce, personalized recommendations play a crucial role in driving customer engagement and increasing sales. Amazon, for example, utilizes collaborative filtering and item-based recommendations to offer users personalized product suggestions. These recommendations are based on user browsing and purchase history, as well as other users’ behavior with similar preferences.
Moreover, some e-commerce platforms go beyond product recommendations and provide personalized styling advice. By considering user preferences, body type, and fashion trends, these platforms offer tailored outfit suggestions, helping users make fashion choices that align with their individual taste.
7. The Future of AI-Powered Assistance
The future of AI-powered assistance holds even more exciting possibilities. As AI technology advances, recommendation systems will continue to improve in accuracy, personalization, and contextual understanding. Natural Language Processing (NLP) will enable voice-based personalized recommendations, making interactions with AI assistants more conversational and intuitive.
Furthermore, the integration of AI-powered assistance in various industries such as healthcare, finance, and education will redefine how personalized recommendations are utilized. From personalized treatment plans to financial advice and customized learning paths, AI-powered assistance will revolutionize the way we receive tailored recommendations and guidance.
FAQs
1. Can AI-powered recommendation systems be biased?
Yes, AI-powered recommendation systems can be biased if the underlying algorithms are not designed or trained properly. Bias can be introduced when the training data is incomplete or biased itself. It is crucial to ensure diverse and representative training data and regularly evaluate and mitigate bias in recommendation systems.
2. How do recommendation systems handle privacy concerns?
Recommendation systems should prioritize user privacy and data protection. Companies should inform users about the data collected, how it will be used, and obtain consent. Implementing anonymization techniques and secure data storage can also help address privacy concerns.
3. Can personalized recommendations result in information overload?
While personalized recommendations aim to reduce information overload, excessive personalization and lack of serendipity might result in creating filter bubbles and limiting exposure to diverse content. Balancing personalization with occasional recommendations outside the user’s comfort zone can help overcome information overload.
References
1. Netflix: Providing Personalized Recommendations Using Machine Learning, https://www.netflix.com
2. Amazon Personalize: Real-Time Personalization and Recommendation Service, https://aws.amazon.com/personalize/
3. Resnick, P., & Varian, H. R. (1997). Recommender systems. Communications of the ACM, 40(3), 56-58.