Welcome to Kodezi AI’s comprehensive guide on mastering complex algorithms! Whether you are a beginner or an experienced AI enthusiast, this guide will provide you with the essential knowledge and techniques to tackle intricate algorithms with confidence.
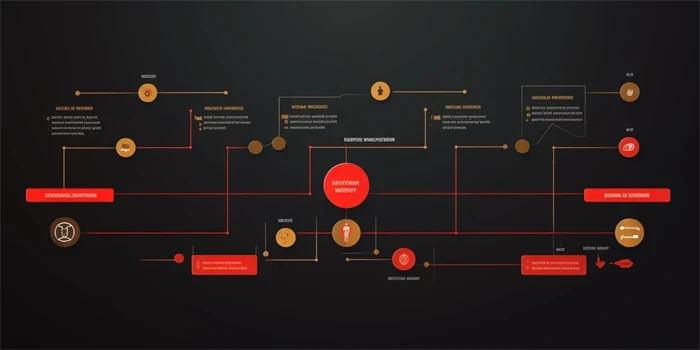
1. Understanding Algorithm Design
Algorithm design is at the core of solving complex problems in AI. It involves breaking down problems into smaller sub-problems, identifying efficient data structures, and creating step-by-step instructions to solve them. A well-designed algorithm ensures optimal performance and reliable results.
Key areas to focus on:
- Analyzing problem complexity
- Determining appropriate data structures
- Developing algorithmic thinking skills
2. Sorting Algorithms Demystified
Sorting algorithms are essential in AI applications like recommendation systems and search algorithms. Understanding and implementing efficient sorting algorithms can significantly improve the performance of your AI models. This section will cover popular sorting techniques such as Bubble Sort, Quick Sort, and Merge Sort, along with their time and space complexities.
3. Searching Algorithms Unveiled
Searching algorithms play a crucial role in AI applications such as natural language processing and information retrieval. We will delve into fundamental searching algorithms, including Linear Search, Binary Search, and Hashing, and explore their strengths and weaknesses.
4. Graph Algorithms for Network Analysis
Graph algorithms are widely used in AI for network analysis, social network analysis, and recommendation systems. We will explore graph traversal algorithms like Breadth-First Search (BFS) and Depth-First Search (DFS), as well as advanced techniques like Dijkstra’s algorithm and Bellman-Ford algorithm for solving shortest path problems.
5. Divide and Conquer Strategy
The divide and conquer strategy is pivotal in designing efficient algorithms. This section will explain the concept of breaking down complex problems into smaller, manageable sub-problems, and illustrate its application with well-known algorithms like Merge Sort and Strassen’s Matrix Multiplication.
6. Dynamic Programming for Optimal Solutions
Dynamic programming is a powerful technique for solving optimization problems. We will explore how to identify subproblems, define recurrence relations, and utilize memoization to efficiently solve problems. The famous examples of dynamic programming include the Knapsack problem and the Fibonacci sequence.
7. Probability and Randomized Algorithms
Probability theory provides essential tools for analyzing randomized algorithms. In this section, we will delve into the basics of probability theory and explore how it applies to algorithms like random selection, Monte Carlo simulation, and randomized quicksort.
8. Application of AI in Algorithm Design
This section will highlight how AI itself can assist in algorithm design. We will discuss techniques such as genetic algorithms, simulated annealing, and reinforcement learning that aid in automating the process of creating efficient algorithms.
Frequently Asked Questions:
- Q: Can I apply these algorithms to real-world AI projects?
- Q: Are there any tools or libraries that can assist in algorithm design?
- Q: How important is algorithm efficiency in AI?
A: Absolutely! The algorithms covered in this guide have widespread application in various AI domains. Understanding and implementing them will undoubtedly enhance your AI project capabilities.
A: Yes, several tools and libraries, such as NumPy, SciPy, and TensorFlow, provide extensive support for implementing complex algorithms in AI projects. These tools offer efficient data structures and functions for effective algorithm design.
A: Algorithm efficiency is paramount in AI applications, especially when dealing with large datasets or time-sensitive tasks. Efficient algorithms minimize processing time, memory usage, and computational resources, resulting in faster and more accurate AI models.
References:
1. Cormen, Thomas H., et al. “Introduction to Algorithms.” MIT Press, 2009.
2. Kleinberg, Jon, and éva Tardos. “Algorithm Design.” Pearson/Addison Wesley, 2005.
3. Sedgewick, Robert, and Kevin Wayne. “Algorithms.” Addison-Wesley Professional, 2011.