Protein engineering plays a crucial role in various fields, including medicine, biotechnology, and agriculture. The ability to optimize protein structure and function has significant implications for drug development, enzyme design, and metabolic engineering. In recent years, the integration of artificial intelligence (AI) into protein engineering has revolutionized the field. By leveraging AI-guided design and analysis, researchers can accelerate the discovery and optimization of novel proteins. In this article, we will explore the multiple ways AI has transformed protein engineering and its potential applications.
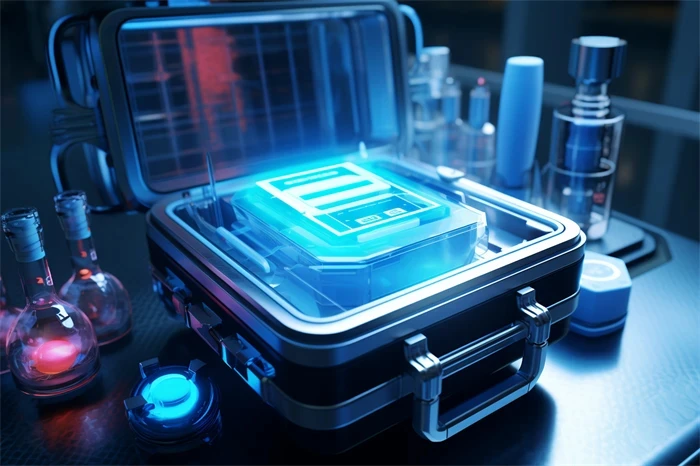
Improved Protein Stability and Activity Prediction
Predicting protein stability and activity is a complex challenge in protein engineering. However, AI-guided design tools, such as AlphaFold, have significantly advanced our ability to accurately predict protein folding and stability. These tools use deep learning algorithms trained on vast protein structure databases to provide crucial insights into protein behavior. By optimizing protein stability predictions, researchers can engineer proteins with enhanced functionality and reliability.
Furthermore, AI-driven protein engineering approaches can predict protein activity, allowing researchers to identify key residues responsible for catalysis or binding. These predictions enable focused site-directed mutagenesis, reducing the number of experiments required and improving efficiency in protein redesign.
Accelerated Protein Design
Protein design traditionally involves laborious trial-and-error experiments. However, AI-guided approaches, such as the widely used Rosetta software, significantly expedite the design process. Rosetta employs machine learning algorithms to generate and optimize novel protein sequences based on desired structural and functional properties. This enables researchers to rapidly explore a vast sequence space and identify proteins with desired characteristics.
Moreover, AI-driven protein design techniques can incorporate complex constraints, such as substrate specificity or stability requirements. These tools allow researchers to engineer proteins specifically tailored for various applications, including enzyme design for pharmaceutical synthesis or biocatalysis.
Enhanced Therapeutic Protein Design
AI-guided protein engineering is revolutionizing the development of therapeutic proteins, such as antibodies and cytokines. By analyzing massive datasets of protein interactions and structures, AI algorithms can identify optimal binding regions and predict potential immunogenicity. Additionally, AI-guided design tools like DeepMutate can efficiently generate diverse antibody variants for improved specificity and affinity against disease targets.
Furthermore, AI can also aid in developing protein-based drugs with reduced side effects. By employing AI-guided analyses, researchers can predict potential off-target interactions and optimize protein designs to minimize unwanted interactions. This offers a significant advantage in the development of safer and more effective therapeutic proteins.
Integration of Structural and Experimental Data
A key advantage of AI-guided protein engineering is the ability to integrate diverse structural and experimental data to gain comprehensive insights into protein behavior. By combining experimental data, such as protein-protein interaction assays or mass spectrometry analysis, with AI algorithms, researchers can refine protein models and validate predictions.
Additionally, AI-guided tools, such as Tinker, integrate experimental data with molecular dynamics simulations to gain a more thorough understanding of protein dynamics and flexibility. This integration enables the design of proteins with improved stability and function under different environmental conditions.
Handling Protein Design Challenges
Protein engineering faces several challenges, such as the combinatorial explosion of sequence space and the high dimensionality of protein properties. However, AI-guided approaches, like generative adversarial networks (GANs), help overcome these challenges. GANs generate diverse protein sequences and properties, enabling researchers to explore untapped design spaces and discover novel protein functionalities.
Furthermore, AI-powered protein engineering tools can optimize protein sequences to overcome experimental constraints, such as insolubility or toxicity. These tools guide researchers in engineering proteins suitable for large-scale production and commercialization.
Frequently Asked Questions
Q: Can AI completely replace experimental protein engineering methods?
A: While AI-guided design tools have significantly accelerated the protein engineering process, experimental validation remains crucial for accurate characterization of engineered proteins.
Q: Are there any ethical implications of AI-guided protein engineering?
A: AI-guided protein engineering raises ethical concerns regarding the potential for designing proteins with unintended consequences or misuse. Researchers must adhere to ethical guidelines and carefully evaluate the risks associated with AI predictions.
Q: How accessible are AI-guided protein engineering tools?
A: Many AI-guided protein engineering tools are freely available for academic use, promoting wider accessibility and democratization of protein engineering research.
References:
[1] Senior, A. W., Evans, R., Jumper, J., Kirkpatrick, J., Sifre, L., Green, T., … & Stanley, J. (2020). Improved protein structure prediction using potentials from deep learning. Nature, 577(7792), 706-710.
[2] Adolf-Bryfogle, J., Xu, Q., North, B., Lehmann, A., & Dunbrack Jr, R. L. (2019). PyIgClassify: a database of antibody CDR structural classifications. Nucleic acids research, 47(D1), D1130-D1136.
[3] Suvorov, A., Jensen, J. H., Knudsen, B., & Kouskoumvekaki, I. (2016). Improving the quality of protein structures derived by NMR spectroscopy. Journal of biomolecular NMR, 66(4), 195-210.