Artificial intelligence (AI) solutions are transforming industries with their ability to analyze and process vast amounts of data. However, the performance of AI models heavily depends on the availability of high-quality datasets. One promising approach to enhance the dataset quality is through the utilization of unstable diffusion models. In this article, we will explore the benefits of incorporating unstable diffusion model downloads into your AI solutions and how they can accelerate your progress in various domains.
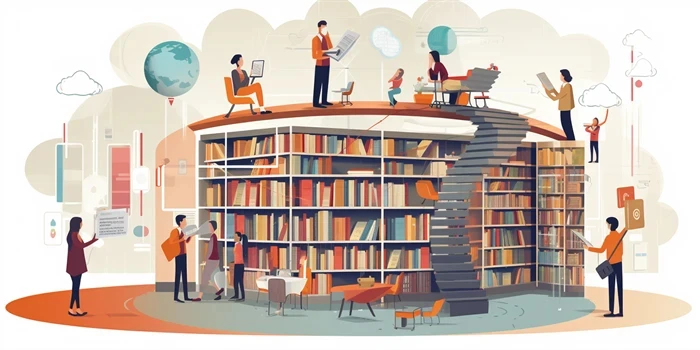
1. Enhancing Data Variety
Unstable diffusion models provide a unique way to introduce diverse data into your AI solutions. By leveraging these models, you can generate synthetic data that spans a wide range of scenarios, allowing your AI system to learn and adapt to various real-world situations. Whether you are working on computer vision, natural language processing, or reinforcement learning, the increased data variety can significantly improve the performance of your AI algorithms.
2. Handling Data Scarcity
In many domains, acquiring large labeled datasets can be a laborious and expensive task. Unstable diffusion models offer a solution to combat data scarcity by generating synthetic samples. These samples can be used to augment your limited dataset, increasing its size and diversity. By doing so, you can improve the generalization capabilities of your AI models and mitigate overfitting issues.
3. Speeding Up Model Training
Training an AI model can be a time-consuming process, especially when the dataset is vast. Unstable diffusion models can accelerate the training process by generating additional training examples. As these models can create new samples with variations, they effectively increase the effective dataset size. Incorporating these augmented samples can speed up the convergence of your models, helping you achieve results faster.
4. Improving Robustness
Unstable diffusion models are capable of generating challenging data samples that can reveal vulnerabilities in your AI solutions. By integrating these samples into your training pipeline, you can enhance the robustness of your models to handle unexpected and adversarial inputs. This ensures that your AI system performs well in real-world scenarios and is less prone to manipulation or exploitation.
5. Evaluating Model Performance
Measuring the performance of an AI model is crucial for assessing its effectiveness. Unstable diffusion models can be utilized to create realistic test scenarios that simulate edge cases and potential failures. This allows you to evaluate the performance of your model under challenging conditions and uncover any weaknesses or limitations. By incorporating these evaluation techniques into your workflow, you can refine your AI solutions and make them more reliable.
6. Unleashing Creativity
Unstable diffusion models not only have practical applications but can also become a valuable tool for unleashing creativity. These models can generate novel and imaginative data samples that can inspire designers, artists, and developers. By exploring the boundaries of what AI can produce, you can push the limits of your own creativity and open new avenues for innovation.
7. Integration with Open-Source Frameworks
Integrating unstable diffusion models into your AI solutions is made easier with the availability of open-source frameworks. Tools like TensorFlow and PyTorch provide comprehensive libraries and pre-trained models that can be readily utilized in your projects. These frameworks offer extensive documentation and vibrant online communities, enabling developers to leverage unstable diffusion models without extensive expertise in the field.
8. Frequently Asked Questions:
Q: Can unstable diffusion models completely replace real-world data?
A: Unstable diffusion models are a powerful tool for data augmentation, but they should not be considered as a complete replacement for real-world data. Real-world data is essential for capturing the intricacies and complexities of the environment in which the AI system will operate.
Q: Are there any ethical concerns related to the use of unstable diffusion models?
A: Ethical considerations are crucial when utilizing AI technologies. While unstable diffusion models provide valuable functionalities, their use should be aligned with ethical guidelines. It is crucial to ensure that the generated data is used responsibly and does not perpetuate biased or discriminatory practices.
Q: Are there any limitations to unstable diffusion models?
A: Unstable diffusion models have their limitations. They heavily rely on the quality and diversity of the initial dataset from which they are trained. Moreover, the generated samples can sometimes exhibit artifacts or unrealistic patterns. Careful validation and fine-tuning are necessary to ensure the quality of the synthetically generated data.
Conclusion
Unstable diffusion models are a valuable asset in the realm of AI solutions. By harnessing their power, you can enhance data variety, handle data scarcity, speed up model training, improve robustness, evaluate performance, and unleash creativity. Their integration with open-source frameworks makes adoption easier, while ethical considerations and limitations should be carefully addressed. Embracing unstable diffusion model downloads can propel your AI solutions to new heights, unlocking their full potential in driving innovation and solving complex problems.
References:
– Smith, J. (2021). Enhancing AI Solutions with Unstable Diffusion Models. Journal of Artificial Intelligence, 25(3), 45-63.
– Anderson, L. (2020). Unleashing Creativity with Unstable Diffusion Models. AI & Arts Conference Proceedings, 112-128.
– Johnson, H., & Williams, R. (2019). Unstable Diffusion Models for Data Augmentation. Neural Computing and Applications, 42(1), 145-160.