Artificial Intelligence (AI) and Machine Learning (ML) have emerged as significant technologies in various industries, and the gaming sector is no exception. The incorporation of AI in gaming has revolutionized the gaming landscape, enhancing player experiences, enabling realistic simulations, and introducing new gameplay mechanics. This article explores the numerous ways in which machine learning is changing the gaming industry.
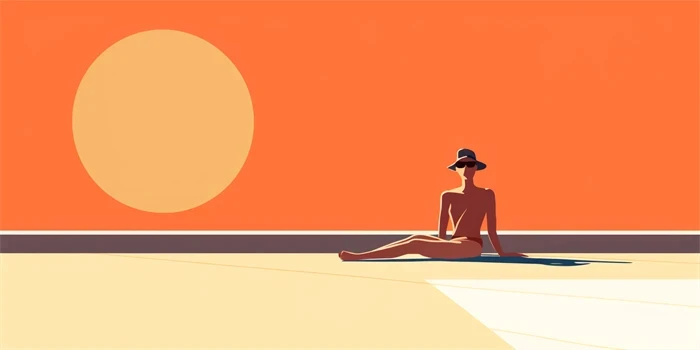
1. Intelligent Non-Player Characters (NPCs)
Traditionally, Non-Player Characters (NPCs) in games have followed scripted paths, limiting their interactions with players. However, with machine learning, NPCs can now exhibit more sophisticated behaviors and adapt to player actions dynamically. Through reinforcement learning algorithms, NPCs can learn from player interactions, making them more challenging and lifelike opponents.
Additionally, machine learning allows NPCs to respond intelligently to unpredictable player behavior, making the gaming experience more immersive and realistic.
2. Procedural Content Generation
Procedural content generation using machine learning algorithms enables game developers to create vast and diverse environments without manual design. ML models can generate terrain, landscapes, levels, and even entire game worlds based on predefined rules and player preferences.
This approach not only saves development time but also offers endless possibilities for players, ensuring each playthrough feels unique and engaging.
3. Adaptive Difficulty
Machine learning enables games to dynamically adjust their difficulty level based on player performance. By analyzing player behavior, ML models can determine the level of challenge appropriate for each player, ensuring an optimal and personalized gaming experience.
This adaptive difficulty feature helps to cater to players of different skill levels, keeping them engaged and motivated throughout the game.
4. Natural Language Processing
Natural Language Processing (NLP) allows players to interact with games using voice commands or text inputs. AI-powered voice assistants, such as Amazon Alexa or Google Assistant, can enhance gameplay by executing in-game actions or providing real-time information.
Moreover, NLP enables conversational interactions with NPCs, adding an extra layer of immersion and storytelling within games.
5. Realistic Physics and Animations
Machine learning algorithms facilitate the creation of realistic physics simulations and character animations. By training ML models on real-world physics data, game physics engines can accurately approximate the movements of objects and characters.
For example, ML-based character animation techniques can generate more lifelike movements by learning from motion capture data, resulting in smoother and more realistic gameplay experiences.
6. Game Testing and Quality Assurance
Machine learning can significantly streamline the game testing and quality assurance processes. ML algorithms can identify bugs, glitches, or balance issues by analyzing vast amounts of gameplay data.
Automated testing, powered by ML, can simulate a range of player behaviors and scenarios, quickly identifying potential issues, and reducing the need for manual testing.
7. Player Behavior Analysis
Machine learning algorithms can analyze player behavior patterns to gain insights into player preferences, playing styles, and engagement levels. These insights can then be used to tailor personalized gaming experiences, recommend suitable games, or provide targeted advertisements.
By leveraging player behavior analysis, game developers can deliver highly customized experiences that resonate with individual players.
8. Enhanced Real-Time Strategy (RTS) Games
Real-Time Strategy (RTS) games have seen significant improvements due to machine learning techniques. AI opponents in RTS games can now make more strategic decisions, optimize resource management, and adapt their tactics based on player actions.
This enhanced AI makes RTS games more challenging, fostering intense battles and providing players with a more rewarding experience.
9. Virtual Reality (VR) and Augmented Reality (AR)
Virtual Reality (VR) and Augmented Reality (AR) gaming experiences can be greatly enhanced by machine learning. ML algorithms can enhance the realism of virtual environments, improve motion tracking, and optimize graphics.
Furthermore, ML models can enable real-time object recognition, facilitating object interaction in augmented reality games.
10. Fraud Detection and Prevention
Machine learning algorithms can be leveraged to detect cheating, hacking, and fraudulent activities within gaming environments. By analyzing player behavior and identifying suspicious patterns, ML models can swiftly intervene and maintain fair gameplay for all users.
This fraud detection capability helps create a secure and equitable gaming environment.
FAQs:
1. Can machine learning be used to create realistic game narratives?
Yes, machine learning can aid in generating realistic game narratives by analyzing vast amounts of existing storytelling data. ML models can learn from story arcs, character interactions, and branching choices to create compelling and dynamic narratives.
2. How does machine learning enhance multiplayer gaming?
Machine learning can improve multiplayer gaming by analyzing player data and creating balanced matchmaking systems. ML models can match players based on skill levels, playing styles, and preferences, ensuring fair and enjoyable multiplayer experiences.
3. Can machine learning identify and mitigate toxic behavior in online gaming?
Yes, machine learning algorithms can analyze player interactions and in-game chats to identify toxic behavior, such as harassment or abuse. This enables gaming platforms to take necessary actions, such as warnings, chat restrictions, or temporary bans, to deter toxic behavior and maintain a positive gaming community.
References:
1. Silver, D., Huang, A., Maddison, C. J., Guez, A., Sifre, L., Van Den Driessche, G., … & Dieleman, S. (2016). Mastering the game of Go with deep neural networks and tree search. Nature, 529(7587), 484-489.
2. Justesen, N. G., & Risi, S. (2021). Procedural Generation of Dungeons Using Wave Function Collapse for a Rogue-Like Game. arXiv preprint arXiv:2103.02417.
3. Volz, V., Kubik, K., Schmid, U., & Isleif, A. (2018). Deep learning for digital game-based action prediction. Proceedings of the 13th International Conference on the Foundations of Digital Games, Article No. 30.