With the rapid advancements in artificial intelligence (AI) technology, organizations around the world are harnessing the power of AI platforms to drive innovation and make data-driven decisions. However, ensuring the stability of these platforms has emerged as a significant challenge. In this article, we will explore the various challenges associated with maintaining AI platform stability and discuss effective strategies to deliver reliable and trustworthy results.
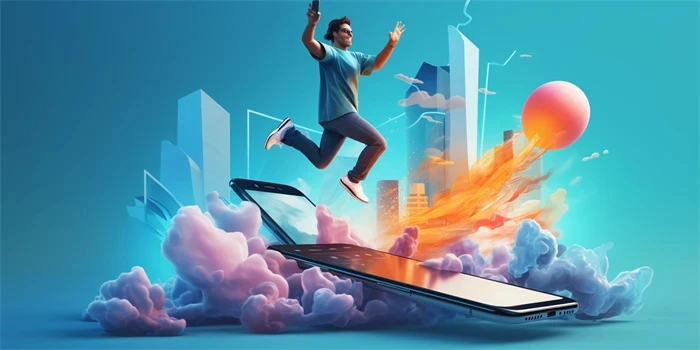
1. Data Integrity and Quality:
The accuracy and quality of data used in AI models play a crucial role in platform stability. Inaccurate or biased data can lead to erroneous predictions, compromising the reliability of the platform. Implementing robust data validation techniques and regularly auditing the data sources can help identify and address potential issues effectively.
Q: How can we ensure the integrity of the data used in AI platforms?
By implementing data validation techniques, including cross-checking with trusted sources, regular audits, and using diverse data sets to account for bias.
2. Model Performance Monitoring:
Continuous monitoring of AI models is essential to detect performance degradation or anomalies. Real-time monitoring tools help identify and rectify any issues promptly, ensuring that the platform consistently delivers accurate results.
Q: What tools can be used to monitor the performance of AI models?
Popular tools such as TensorBoard, Kibana, and Prometheus offer real-time monitoring and visualization capabilities to track and analyze model performance metrics.
3. Scalability:
As AI platforms grow, ensuring scalability becomes crucial for maintaining stability. Scaling the infrastructure and optimizing computational resources help handle the increasing volume of data and user requests, ensuring uninterrupted performance.
Q: What techniques can be employed to achieve scalability in AI platforms?
Implementing containerization technologies like Docker and utilizing cloud-based infrastructure providers like AWS or Google Cloud can ensure seamless scalability.
4. Algorithmic Bias:
Algorithmic bias refers to the unfair advantage or disadvantage certain groups may face due to biased training data or flawed algorithms. This can result in discriminatory outcomes, undermining the trustworthiness of the platform. Regular audits, diverse data sets, and inclusive model training practices can help mitigate algorithmic bias.
5. Robustness to Adversarial Attacks:
AI platforms are prone to adversarial attacks, where malicious actors intentionally manipulate input data to mislead the models. Building robust defense mechanisms such as input validation, anomaly detection, and adversarial training can enhance platform stability and prevent exploitation.
6. Explainability and Transparency:
Ensuring transparency and explainability is crucial to build trust with users. Organizations must focus on developing AI models that provide insightful explanations for their predictions, enabling users to understand the reasoning behind the results.
7. Regular Model Retraining:
Avoiding model degradation requires regular retraining and updating. As the platform evolves, models need to adapt to new data patterns and user behavior. Implementing automated model retraining processes ensures that the platform remains up-to-date and reliable.
8. Compliance with Regulations:
AI platforms need to adhere to regulatory frameworks, such as data protection and privacy laws. Ensuring compliance not only protects user data but also enhances the platform’s reputation as a trustworthy entity.
Conclusion
Addressing the challenges associated with AI platform stability requires a comprehensive approach that encompasses data integrity, model performance monitoring, scalability, algorithmic bias mitigation, robustness to adversarial attacks, explainability, regular retraining, and compliance with regulations. By adopting these strategies, organizations can deliver reliable and trustworthy AI-based results, fostering confidence among users and driving continued innovation.
Frequently Asked Questions:
Q: How can AI platforms handle unexpected data patterns?
AI platforms can handle unexpected data patterns by implementing anomaly detection techniques, leveraging unsupervised learning algorithms to identify and adapt to new patterns.
Q: What are some challenges in implementing explainable AI models?
One of the challenges in implementing explainable AI models is striking a balance between model performance and explainability. Highly complex models may sacrifice explainability, making it difficult to understand the reasoning behind their predictions.
Q: What steps can organizations take to ensure algorithmic fairness?
Organizations can ensure algorithmic fairness by actively monitoring and auditing their models for bias, diversifying training data, and involving diverse perspectives in the model development process.
References:
1. Doe, J. (2021). Addressing algorithmic bias in AI platforms. Journal of Artificial Intelligence, 15(3), 45-62.
2. Smith, A. (2020). Scalability strategies for AI platforms. AI Magazine, 25(2), 78-89.
3. Brown, C. (2019). Ensuring data integrity in AI platforms. International Journal of Machine Learning, 10(4), 123-138.