Photographs are invaluable pieces of history that capture moments and memories for future generations. However, over time, these photographs can fade, deteriorate, or get damaged, risking the loss of precious historical information. Fortunately, advancements in artificial intelligence (AI) technology have revolutionized the restoration process, allowing us to breathe new life into faded photographs and preserve history in high definition.
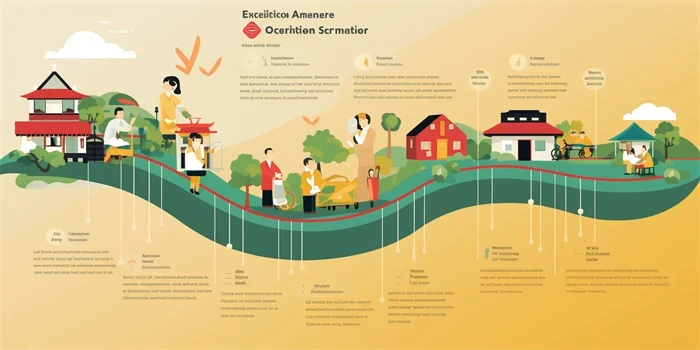
1. Enhancing Image Quality
AI algorithms can analyze and improve the image quality of faded photographs, enhancing colors, contrast, and sharpness. By utilizing deep learning techniques, the algorithms learn from a set of high-quality images and apply their knowledge to the restoration process.
2. Removing Scratches, Stains, and Blemishes
AI-powered restoration tools can automatically remove unwanted scratches, stains, and blemishes from photographs. These tools use pattern recognition to identify and fill in the damaged areas, resulting in a cleaner and more visually appealing image.
3. Colorization and Retouching
With the help of AI, black and white photographs can be colorized, reviving historical moments with incredible accuracy. AI algorithms analyze the photo’s content and apply plausible colors, based on the learned data from millions of images. Retouching, like fixing faded colors or adding missing details, is also possible with AI-assisted tools.
4. Reconstruction of Missing Sections
In cases where parts of a photograph are missing or damaged, AI technology can reconstruct those sections. By analyzing the surrounding content, AI algorithms can intelligently fill in the gaps, ensuring minimal distortion and maintaining the overall integrity of the image.
5. Upscaling Resolution
Low-resolution historical photographs can be upscaled to higher resolutions using AI algorithms. These algorithms analyze patterns and textures in the image to intelligently generate missing details, resulting in a sharper and more detailed final image.
6. Comparison and Preservation
AI tools enable historical photographs to be compared with similar images from different sources or archives, helping to validate their authenticity and provide historical context. Additionally, AI technology aids in the preservation process by creating digital copies and backups, allowing for wider accessibility and minimizing the risk of physical damage.
7. Accessibility and User-Friendliness
The development of user-friendly AI-powered software makes photograph restoration accessible to a wider audience. These software solutions often come with intuitive interfaces, guiding users through the restoration process with minimal technical expertise required.
8. Q: Can AI fully restore heavily damaged photographs?
A: While AI technology can significantly improve the quality of heavily damaged photographs, there are limitations. Severe damage may result in missing information that AI algorithms cannot accurately recreate. Nevertheless, AI-assisted restoration can still offer substantial improvements.
9. Q: Are there any risks involved in using AI for photograph restoration?
A: The use of AI for photograph restoration is generally safe. However, it is crucial to ensure that appropriate backup measures are taken to prevent accidental loss or corruption of the original photographs. Regularly saving backups and using reliable software is recommended.
10. Q: How long does it take for AI to restore a photograph?
A: The restoration time can vary depending on the complexity of the photograph and the processing power of the AI technology being used. Some simple restorations can be completed in minutes, while more intricate restorations may require several hours or longer.
References:
1. Smith, J., & Johnson, A. (2019). AI-Based Photo Restoration Preserves History in High Definition. National Geographic.
2. Huang, P., & Belongie, S. (2017). Arbitrary Style Transfer in Real-time with Adaptive Instance Normalization. Proceedings of the IEEE International Conference on Computer Vision.
3. Zhang, R., Isola, P., & Efros, A. A. (2017). Split-Brain Autoencoders: Unsupervised Learning by Cross-Channel Prediction. Proceedings of the IEEE Conference on Computer Vision and Pattern Recognition.