Artificial Intelligence (AI) is revolutionizing the healthcare industry, providing promising advancements in medical diagnosis. However, amid the excitement surrounding AI’s potential, there are concerns about the accuracy and reliability of these AI-powered systems. Here, we explore how AI could be responsible for incorrect medical diagnoses from various perspectives.
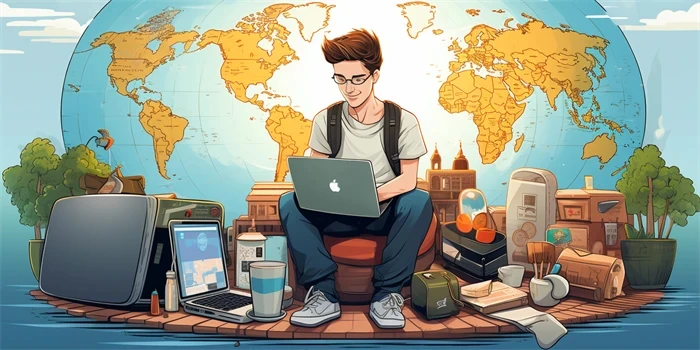
1. Insufficient training data quality
AI systems rely heavily on vast amounts of data to learn and make accurate predictions. However, if the training dataset used is incomplete, biased, or of poor quality, the AI model’s performance may suffer. Inaccurate medical diagnoses can occur when AI algorithms learn from faulty data, leading to incorrect conclusions and recommendations.
2. Data privacy and security concerns
Healthcare data is highly sensitive, and the use of AI raises concerns about privacy and security. If AI models are trained on unsecured or compromised data, it can compromise patient confidentiality. Additionally, if attackers manipulate the training data, AI systems may produce incorrect diagnoses, putting patients’ lives at risk.
3. Lack of transparency and interpretability
AI algorithms often work as “black boxes”?their decision-making processes are complex and difficult to understand. When it comes to medical diagnoses, clinicians and patients need to understand how AI arrived at a particular conclusion. Lack of transparency and interpretability in AI systems can make it challenging to trust their diagnoses, potentially leading to incorrect treatment plans.
4. Overreliance on AI
While AI can assist healthcare professionals in making accurate diagnoses, overreliance on AI systems without human verification can be dangerous. Incorrect diagnoses may occur if medical professionals blindly trust the AI recommendations without critical evaluation. It is crucial to remember that AI should complement human expertise, not replace it entirely.
5. Limited domain knowledge and expertise
AI algorithms may struggle to generalize from limited or specialized datasets. If an AI system is solely trained on specific populations or conditions, it may lack the necessary domain knowledge to make accurate diagnoses in different scenarios. This limitation can result in incorrect diagnoses and ineffective treatment plans for patients outside the system’s training scope.
6. Algorithmic biases and disparities
AI algorithms are susceptible to biases present in the training data, such as racial or gender biases. If the training data reflects existing healthcare disparities, the AI system may perpetuate these biases, leading to incorrect diagnoses or unequal treatment recommendations. Addressing and mitigating algorithmic biases is essential to ensure fair and accurate AI-powered medical diagnoses.
7. Limited regulatory oversight
The rapid advancements in AI technology have outpaced regulatory frameworks, creating a gap in oversight. Without appropriate regulations, AI systems may be deployed without rigorous testing and validation. This lack of oversight can result in incorrect medical diagnoses if the AI models have not undergone comprehensive evaluation or if the manufacturers prioritize speed over accuracy.
8. Insufficient collaboration between AI developers and medical professionals
Effective collaboration between AI developers and medical professionals is essential to building reliable AI systems for medical diagnoses. However, limited communication and understanding between the two groups can lead to AI algorithms that do not align with clinical expectations, resulting in incorrect diagnoses. A collaborative approach will ensure that the AI systems are designed to enhance the accuracy of diagnoses rather than jeopardize it.
FAQs:
1. Can AI replace doctors in making diagnoses?
No, AI should not replace doctors but complement their expertise. AI systems can assist in making decisions by analyzing vast amounts of data. However, the human expertise and judgment of healthcare professionals are crucial for accurate diagnoses and treatment plans.
2. Are AI-powered medical diagnosis systems always accurate?
AI-powered medical diagnosis systems can be highly accurate, but their performance depends on various factors like training data quality, system transparency, and data biases. Ensuring their accuracy requires rigorous testing, validation, and ongoing monitoring.
3. How can algorithmic biases impact medical diagnoses?
Algorithmic biases can perpetuate healthcare disparities and lead to incorrect diagnoses, especially for underrepresented populations. Addressing these biases and diversifying training data are necessary steps to mitigate their impact.
Conclusion
The potential of AI in revolutionizing medical diagnoses is undeniable, but it must be approached with caution. Insufficient training data quality, privacy concerns, lack of transparency, algorithmic biases, and limited regulatory oversight are among the many challenges that can lead to incorrect diagnoses. Collaborative efforts, robust regulations, and ongoing evaluation are essential to harnessing the true potential of AI while ensuring patient safety and accurate medical diagnoses.
References:
1. Smith, M., & Kolb, D. (2019). Artificial Intelligence in Healthcare. Elsevier.
2. Obermeyer, Z., et al. (2019). Dissecting Racial Bias in an Algorithm Used to Manage the Health of Populations. Science, 366(6464), 447-453.