Artificial Intelligence (AI) systems are increasingly being used in decision-making processes across various domains, such as healthcare, financial services, and criminal justice. However, there is a growing concern about the presence of bias and discrimination in these AI models, which can lead to unfair outcomes and perpetuate social inequalities. In this article, we will explore the different facets of bias and discrimination in AI decision-making and discuss strategies to mitigate them.
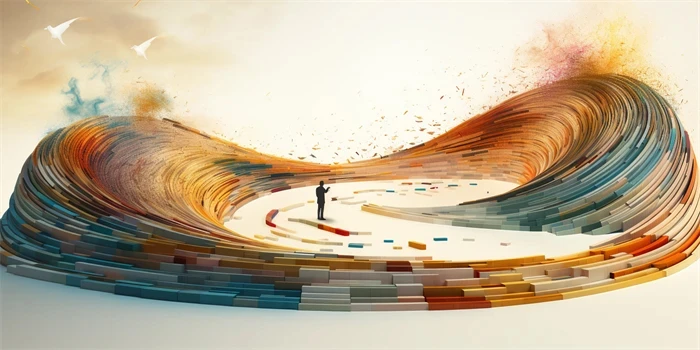
1. Understanding Bias in AI Models
Bias in AI models refers to the systematic errors or prejudices that can occur due to the data used to train these models. It can arise from various sources, including historical biases in the training data, biased labeling, or biased feature selection. To address bias, it is important to conduct a thorough analysis of the training data to identify potential bias sources and take appropriate corrective measures.
2. The Role of Diversity in Training Data
A key factor in reducing bias in AI models is ensuring diversity in the training data. By incorporating a wide range of demographic, socio-economic, and cultural factors, AI models can better reflect the diversity of the population and avoid discriminatory outcomes. However, it is crucial to be cautious while collecting diverse datasets to avoid introducing new biases or perpetuating existing ones.
3. Fairness Metrics and Evaluation
Developing fairness metrics and evaluation methods specific to AI models is essential for assessing their performance in terms of fairness. Various fairness measures, such as disparate impact, equal opportunity, and demographic parity, can be employed to analyze the models’ behavior and identify areas where biases are prevalent. By regularly evaluating AI models against these metrics, developers can track progress and make necessary modifications.
4. Model Explainability and Transparency
Ensuring transparency and explainability of AI models is crucial for identifying and addressing bias. Techniques such as interpretable machine learning can provide insights into the decision-making process of AI models, making it easier to detect and understand biases. Enhancing transparency also fosters accountability and enables affected individuals to contest unfair decisions.
5. Regular Bias Audits and Real-time Monitoring
To maintain fairness in AI decision-making, conducting regular bias audits and implementing real-time monitoring are essential. Bias audits involve continuously assessing the performance of AI models and identifying any potential biases that may have been introduced. Real-time monitoring helps detect and mitigate biases as they emerge, ensuring timely corrective actions.
6. Ethical Considerations in AI Development
Integrating ethical considerations into the development of AI models is fundamental for promoting fairness. Developers should prioritize ensuring that AI models align with societal values and do not perpetuate or amplify existing biases and discrimination. Ethical guidelines and frameworks, such as those proposed by organizations like the Partnership on AI, can serve as valuable resources for designing and evaluating ethical AI systems.
7. Human Oversight and Decision-making
While AI models play a significant role in decision-making, it is crucial to maintain human oversight and involvement. Human expertise can provide valuable insights, ensure context-aware decision-making, and prevent potential biases from going unnoticed. AI systems should be designed to augment human decision-making rather than replace it entirely.
8. Collaborative Efforts and Knowledge Sharing
Addressing bias and discrimination in AI decision-making requires collaborative efforts from various stakeholders, including researchers, policymakers, industry experts, and affected communities. Knowledge sharing and collaboration between these stakeholders can help identify best practices, share resources, and collectively work towards developing fair and unbiased AI models.
Conclusion
Fostering fairness in AI decision-making is an ongoing challenge, but it is crucial to address biases and discrimination to ensure just outcomes. By understanding the different aspects of bias, promoting diversity, employing fairness metrics, ensuring transparency, conducting regular audits, considering ethics, integrating human oversight, and fostering collaboration, we can take significant steps towards achieving fair AI decision-making and minimizing societal inequities.
Frequently Asked Questions
Q1: Can AI completely eliminate bias in decision-making?
A1: While AI can help mitigate bias, complete elimination is challenging since bias can stem from the underlying data and societal dynamics. However, conscious efforts can be made to reduce bias significantly.
Q2: How can individuals affected by biased AI decisions seek recourse?
A2: It is important to establish channels for individuals to contest AI decisions. This can include creating mechanisms for appeals, providing explanations for decisions, and engaging in dialogues with affected individuals.
Q3: Are bias and discrimination only prevalent in AI decision-making?
A3: Bias and discrimination can exist in various decision-making processes. However, AI has garnered significant attention due to its potential to amplify biases at scale if not appropriately developed and monitored.
References:
[1] Caliskan, A., Bryson, J.J., and Narayanan, A. (2017). Semantics derived automatically from language corpora contain human-like biases. Science, 356(6334), 183-186.
[2] Barocas, S., Hardt, M., and Narayanan, A. (2019). Fairness and Machine Learning. United States: Cambridge University Press.
[3] Partnership on AI. (2020). Ethical Framework for AI in Healthcare. Retrieved from https://www.partnershiponai.org/ai-in-healthcare-ethical-framework/