In recent years, the financial industry has witnessed a significant transformation with the integration of Artificial Intelligence (AI) and Machine Learning (ML) technologies. These advancements have revolutionized how investors make data-driven decisions, optimize portfolios, and manage risks. By leveraging the power of AI and ML, financial institutions have gained a competitive edge in the market while improving efficiency and accuracy. In this article, we will explore the various ways in which AI and ML are being utilized in finance and the benefits they bring.
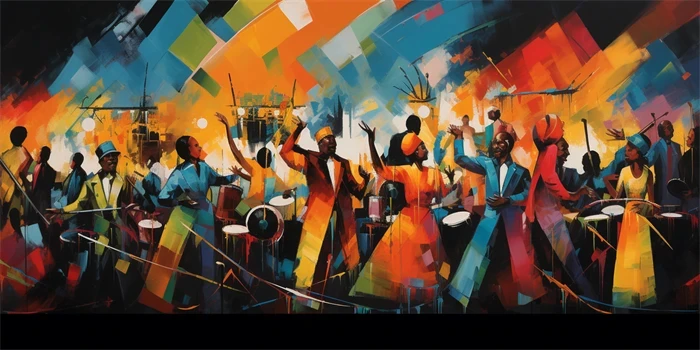
1. Risk Assessment and Management
One of the key applications of AI in finance is risk assessment and management. ML algorithms can analyze vast amounts of historical data to detect patterns and trends, enabling financial institutions to make more informed risk assessments. This helps to identify potential risks, such as credit default or market volatility, and develop appropriate risk management strategies.
Furthermore, AI-powered risk management systems can continuously monitor and analyze real-time data to detect any anomalies or deviations from normal patterns. This enables timely intervention to mitigate potential risks and prevents substantial financial losses.
2. Fraud Detection and Prevention
AI has proven to be highly effective in detecting and preventing fraud in the financial sector. ML algorithms can analyze large datasets to identify unusual patterns or behaviors that may indicate fraudulent activity. This includes analyzing transactional data, customer behavior, and network connections to detect anomalies.
Moreover, AI can continuously learn and adapt to new fraud techniques, making it more robust and accurate over time. By leveraging AI for fraud detection, financial institutions can save millions of dollars in losses and protect their customers’ finances.
3. Trading and Investment Strategies
Machine Learning plays a crucial role in developing trading and investment strategies. ML algorithms can analyze historical market data, news sentiment, and other relevant factors to identify profitable trading opportunities. By utilizing AI, traders can make more informed decisions on when to buy, sell, or hold financial instruments.
Furthermore, AI-powered trading systems can react to market movements in real-time, enabling high-frequency trading and taking advantage of short-lived opportunities. This automation reduces human error and ensures faster execution, resulting in improved investment returns.
4. Customer Service and Personalization
AI-powered chatbots and virtual assistants have revolutionized customer service in the financial industry. These intelligent systems can answer customer queries, provide account information, and assist with basic transactions. Through Natural Language Processing (NLP) capabilities, chatbots can understand and respond to customer inquiries in a human-like manner.
Additionally, ML algorithms can analyze customer data to provide personalized recommendations and financial advice. This enhances customer experience and helps financial institutions build stronger relationships with their clients.
5. Credit Scoring and Loan Underwriting
Traditional credit scoring models often rely on limited information and may not accurately assess an individual’s creditworthiness. AI and ML algorithms can analyze a broader range of data, including alternative data sources such as social media profiles or transaction history, to provide more accurate credit scores.
Furthermore, AI-powered loan underwriting systems can automate the loan approval process by analyzing various factors such as income, employment history, and debt-to-income ratio. This reduces the time taken for loan processing and improves efficiency, benefitting both lenders and borrowers.
6. Market Research and Investment Analysis
AI and ML technologies have significantly enhanced market research and investment analysis. These tools can analyze an extensive range of data, including financial statements, news articles, social media sentiment, and economic indicators, to identify trends and forecast market movements.
Additionally, AI-powered investment analysis platforms can generate insights and recommendations based on the analysis of vast amounts of data in real-time. This enables investors to make more informed decisions and adapt to changing market conditions swiftly.
7. Regulatory Compliance
A major challenge in the financial industry is complying with complex regulatory frameworks. AI and ML can automate compliance processes, ensuring adherence to regulations while mitigating the risk of human error. These technologies can analyze legal documents, monitor transactions, and identify potential compliance breaches.
By utilizing AI for regulatory compliance, financial institutions can reduce costs associated with manual compliance tasks and improve overall compliance effectiveness.
8. Insurance Underwriting
AI and ML are transforming the insurance industry by automating and improving underwriting processes. ML algorithms can analyze large amounts of data, including historical claims data, medical records, and demographic information, to assess risk accurately and determine appropriate premiums.
Furthermore, AI-powered chatbots and virtual assistants can assist customers with insurance-related queries, claims processing, and policy management, providing a seamless and efficient customer experience.
FAQs
Q: Can AI completely replace human financial advisors?
A: While AI can provide personalized recommendations and analysis, it cannot completely replace human financial advisors. Human advisors bring essential qualities such as empathy, intuition, and the ability to understand complex individual circumstances, which AI may lack.
Q: How does AI help with portfolio optimization?
A: AI algorithms can analyze historical and real-time market data, risk profiles, and investment objectives to optimize portfolio allocations. It considers various factors such as risk tolerance, diversification, and expected returns to create portfolios that align with an investor’s goals.
Q: Is AI biased in its decision-making?
A: AI can be biased if the training data used contains biases or if the algorithms are not designed to adequately address biases. It is crucial to ensure that data used for AI models is diverse and representative to minimize potential biases.