Artificial Intelligence (AI) has revolutionized various industries, enabling machines to mimic human intelligence and perform complex tasks. One of the most important components of AI is deep learning algorithms. These algorithms allow machines to process and analyze vast amounts of data, enabling them to make accurate predictions and decisions. In this article, we will unravel the mysteries of AI by providing a comprehensive understanding of deep learning algorithms.
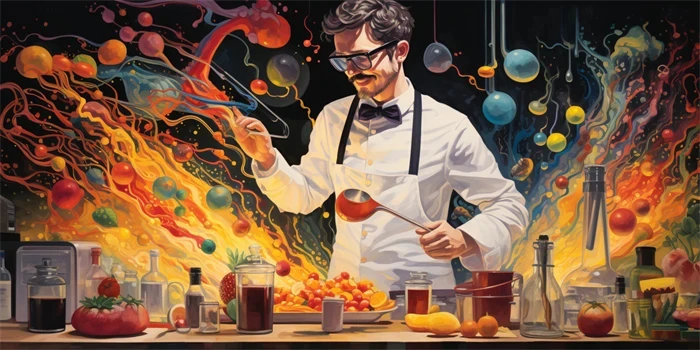
1. Introduction to Deep Learning
Deep learning is a subset of AI that focuses on training neural networks with multiple layers to learn and understand patterns in data. It is inspired by the structure and function of the human brain, where each layer of neurons processes and extracts features from the input data. Through this hierarchical approach, deep learning algorithms can automatically learn representations of data, leading to improved accuracy and performance.
2. Neural Networks and Activation Functions
Neural networks are the backbone of deep learning algorithms. They are composed of interconnected nodes called neurons, and each neuron performs a mathematical operation on the input data. Activation functions, such as sigmoid, ReLU, and tanh, introduce non-linearities to the network, enabling it to capture complex relationships between the input and output.
3. Training a Deep Learning Model
Training a deep learning model involves two key steps: forward propagation and backpropagation. In forward propagation, the input data is passed through the network, and predictions are made at the output layer. Backpropagation is then used to calculate the gradients of the model’s parameters with respect to a loss function. These gradients are used to update the model’s parameters through optimization algorithms like stochastic gradient descent.
4. Convolutional Neural Networks (CNNs)
CNNs are a type of deep learning model specifically designed for analyzing visual data, such as images. They utilize convolutional layers to extract spatial features from the input and pooling layers to reduce the dimensionality of the features. CNNs have revolutionized image recognition tasks and have been successfully applied in various fields, including autonomous driving, medical imaging, and facial recognition.
5. Recurrent Neural Networks (RNNs)
RNNs are neural networks that have connections between neurons forming a directed cycle, allowing them to utilize sequential information. They excel in tasks involving sequential data, such as natural language processing and speech recognition. A popular variant of RNNs is Long Short-Term Memory (LSTM), which addresses the vanishing gradient problem and preserves long-term dependencies in the data.
6. Generative Adversarial Networks (GANs)
GANs are a class of deep learning models that consist of two components: a generator and a discriminator. The generator generates synthetic data, while the discriminator distinguishes between real and fake data. GANs have been used to generate realistic images, create deepfake videos, and enhance data augmentation techniques.
7. Challenges and Limitations of Deep Learning
Despite their impressive capabilities, deep learning algorithms face several challenges and limitations. They require vast amounts of labeled training data, and their training process can be computationally expensive and time-consuming. Additionally, they are susceptible to overfitting if the model is not properly regularized and can struggle with interpretability, making it difficult to understand the decision-making process.
Frequently Asked Questions
Q: What is the difference between AI and deep learning?
A: AI is a broad field encompassing various techniques to enable machines to exhibit human-like intelligence. Deep learning is a subset of AI that focuses on training neural networks with multiple layers to learn and understand patterns in data.
Q: Can deep learning models be easily interpreted?
A: Deep learning models often lack interpretability, making it challenging to understand how they arrive at their decisions. Research is ongoing to develop techniques for explaining and interpreting deep learning models.
Q: How much data is required to train a deep learning model?
A: Deep learning models typically require a large amount of labeled training data to achieve good performance. However, techniques such as transfer learning and data augmentation can alleviate the data requirements.
References
1. LeCun, Y., Bengio, Y., & Hinton, G. (2015). Deep learning. Nature, 521(7553), 436-444.
2. Goodfellow, I., Bengio, Y., & Courville, A. (2016). Deep Learning. MIT Press.
3. Schmidhuber, J. (2015). Deep learning in neural networks: An overview. Neural Networks, 61, 85-117.