Artificial Intelligence (AI) has gained significant attention in recent years due to its vast potential in revolutionizing various industries. Deep Learning, a subset of AI, has emerged as a powerful technique that mimics the human brain’s neural networks. It has paved the way for many groundbreaking applications, such as image recognition, natural language processing, and autonomous driving. In this article, we will delve into the mysteries behind Deep Learning and explore how it unlocks the true potential of AI.
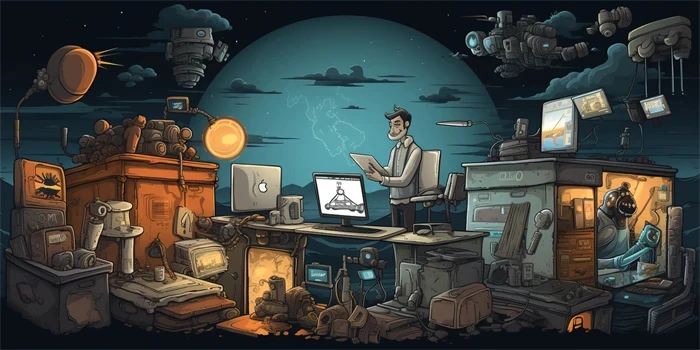
1. Understanding Deep Learning
Deep Learning is a branch of machine learning that aims to train artificial neural networks to perform complex tasks without explicit programming instructions. It is based on the concept of deep neural networks, which consist of multiple layers of interconnected nodes called neurons. These networks learn from large datasets to discover intricate patterns and relationships.
2. The Role of Neural Networks
Neural networks are the backbone of deep learning models, simulating the behavior of human neurons. Each neuron receives inputs, performs calculations, and passes the result to the next layer. This process continues until the final output is generated. The strength of each connection, known as weights, is adjusted during training to optimize the network’s performance.
3. Training Deep Learning Models
To train a deep learning model, a vast amount of labeled data is required. The model learns from the data by adjusting its weights through a process called backpropagation. This iterative process involves comparing the predicted output with the actual output and updating the weights accordingly. The model continues to iterate until it achieves a satisfactory level of accuracy.
4. Importance of Big Data
Deep learning thrives on big data. The availability of enormous datasets enables models to learn intricate patterns and generalize them to new examples. Big data also allows models to account for a wide range of scenarios, leading to robust and reliable predictions.
5. Computing Power and Deep Learning
Deep learning models require substantial computing power, especially when dealing with large datasets. Graphics Processing Units (GPUs) have become a popular choice due to their ability to accelerate matrix operations, which are fundamental to neural network computations. Additionally, cloud computing platforms provide scalable and on-demand resources for training deep learning models.
6. Deep Learning Frameworks and Libraries
There are various deep learning frameworks and libraries available to facilitate the implementation of deep learning models. TensorFlow, developed by Google, is widely used due to its versatility and extensive community support. PyTorch, another popular framework, offers a dynamic computational graph and intuitive design. Choosing the right framework depends on the specific requirements and familiarity of the user.
7. Applications of Deep Learning
Deep Learning has found applications in numerous fields. In healthcare, it aids in medical image analysis, disease diagnosis, and drug discovery. In finance, it assists in fraud detection, stock market analysis, and algorithmic trading. In autonomous driving, deep learning enables vehicles to perceive their surroundings and make informed decisions. The potential applications are vast and continue to expand.
8. Challenges and Limitations
While deep learning has achieved remarkable success, several challenges and limitations persist. Overfitting, where the model memorizes the training data instead of learning general patterns, is a common issue. Data privacy and security concerns arise when dealing with sensitive information. The interpretability of deep learning models is another challenge, as they often operate as black boxes, making it difficult to understand their decision-making process.
9. Frequently Asked Questions
Q: How can deep learning models improve image recognition?
A: Deep learning models can automatically learn high-level features from raw images, enabling them to recognize objects, faces, and scenes more accurately than traditional computer vision techniques.
Q: Can deep learning models understand human language?
A: Yes, deep learning models can process natural language and perform tasks such as sentiment analysis, language translation, and text generation.
Q: Are there ethical concerns regarding the use of deep learning?
A: Yes, ethical concerns such as bias in decision-making, job displacement, and privacy breaches arise with the increasing adoption of deep learning technologies.
Conclusion
Deep learning has unlocked the true potential of AI by enabling machines to learn from vast amounts of data and make accurate predictions. By unraveling the mysteries behind Deep Learning, we have explored its fundamental concepts, applications, challenges, and future prospects. As technology continues to advance, Deep Learning will undoubtedly play a pivotal role in shaping the future of AI and transforming various industries.
References:
1. LeCun, Y., Bengio, Y., & Hinton, G. (2015). Deep learning. Nature, 521(7553), 436-444.
2. Goodfellow, I., Bengio, Y., & Courville, A. (2016). Deep Learning. MIT Press.