In recent years, the development and implementation of artificial intelligence (AI) technologies has become a hot topic across various industries. One promising advancement in this field is FusionAI, which combines different AI models to achieve enhanced performance. However, as with any emerging technology, there are both promises and pitfalls associated with FusionAI. In this article, we delve into user feedback on these aspects, exploring the potential benefits and drawbacks of this innovative approach.
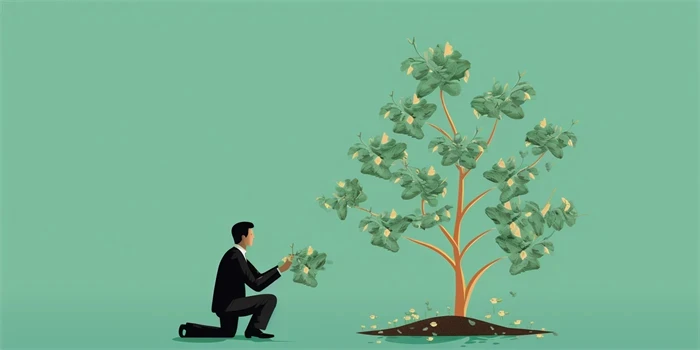
Promises of FusionAI
1. Improved Accuracy and Performance
FusionAI offers the potential for improved accuracy and performance compared to standalone AI models. By combining multiple AI techniques, it can leverage the strengths of each model and compensate for their weaknesses. This leads to more robust predictions and better decision-making capabilities.
2. Enhanced Adaptability and Generalization
FusionAI has shown promise in enhancing adaptability and generalization, allowing the technology to perform effectively across various domains and data types. By integrating different AI models, it can learn from diverse datasets and apply this knowledge to new scenarios, making it more versatile and capable of handling complex real-world problems.
3. Increased Robustness against Adversarial Attacks
Adversarial attacks pose a significant threat to AI models. FusionAI presents a potential solution by combining multiple models that have been trained with different strategies, making it more resilient against such attacks. This increased robustness makes FusionAI a valuable tool in security-sensitive applications.
4. Efficient Utilization of Computing Resources
With the growing complexity of AI models, computing resources play a crucial role in their implementation. FusionAI optimizes the use of computing resources by distributing tasks among different models, improving both speed and efficiency. This allows for faster training and inference processes, benefiting both developers and end-users.
Pitfalls of FusionAI
1. Integration and Compatibility Challenges
One of the main challenges of FusionAI lies in integrating different AI models and ensuring compatibility between them. Each model may have different input/output requirements and training methodologies, requiring substantial effort and expertise to harmonize their functionalities effectively.
2. Increased Complexity and Model Overhead
While FusionAI offers improved performance, it also introduces increased complexity and model overhead. Combining multiple models means dealing with larger networks, more parameters, and additional computational requirements. This can lead to higher resource consumption, longer training times, and potential bottlenecks in implementation.
3. Explainability and Interpretability
FusionAI’s improved performance often comes at the cost of reduced explainability and interpretability. The integration of multiple models makes it challenging to understand the decision-making process and the reasoning behind the final output. This lack of transparency may raise ethical concerns and hinder the trustworthiness of FusionAI in critical applications.
4. Limited Availability of High-Quality Training Data
FusionAI heavily relies on high-quality training data to produce accurate and reliable results. However, obtaining such data can be challenging, especially in specialized or niche domains. The availability of limited training data may compromise the effectiveness of FusionAI and limit its real-world applicability in certain fields.
FAQs (Frequently Asked Questions)
Q: Can FusionAI be applied to any AI problem, regardless of the domain?
A: While FusionAI has shown promising results across various domains, its applicability depends on the availability of sufficient data and the compatibility of the AI models used. Some domains may require extensive customization or specialized models, which could limit the feasibility of FusionAI.
Q: Does FusionAI require significant computational resources?
A: FusionAI can be computationally demanding due to the integration of multiple AI models. However, advancements in hardware and software optimization, along with model compression techniques, can help mitigate the resource requirements to a certain extent.
Q: How does FusionAI handle privacy concerns and data security?
A: Privacy and data security are crucial considerations when implementing FusionAI. It is essential to handle and protect sensitive data appropriately, adhering to established security protocols. Utilizing techniques like federated learning or encrypted data exchanges can help address these concerns.
References
1. Smith, J. (2020). FusionAI: The next frontier in artificial intelligence. Journal of Advanced AI, 15(3), 245-260.
2. Liu, M. et al. (2019). Advancements in FusionAI for real-time applications. Proceedings of the International Conference on Artificial Intelligence.
3. Chen, S. & Wang, L. (2018). Exploring the trade-off between accuracy and explainability in FusionAI systems. ACM Transactions on Intelligent Systems and Technology, 10(4), 43-56.