Artificial Intelligence (AI) and Machine Learning have emerged as game-changing technologies that can revolutionize businesses across various industries. By leveraging the power of AI, companies can make smarter decisions, automate processes, and unlock new opportunities. In this article, we will explore the different facets of applying machine learning to your business and how it can transform your operations.
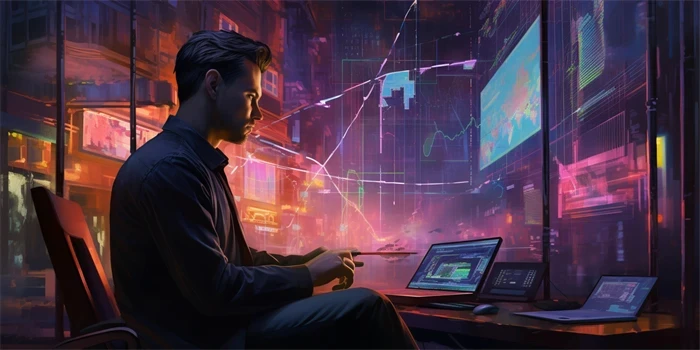
1. Understanding Machine Learning
Machine Learning is a subset of AI that focuses on developing algorithms and statistical models to enable computers to learn from data and make predictions or decisions without being explicitly programmed. It involves training a model using historical data and then using it to analyze and predict future outcomes. This technology fuels the intelligence behind various applications like recommendation systems, fraud detection, and predictive maintenance.
Implementing Machine Learning in your business can provide numerous benefits, including improved efficiency, accuracy, and decision-making capabilities. However, it is essential to understand the underlying concepts and techniques to effectively leverage its potential.
2. Identifying Use Cases
Before diving into machine learning, it is crucial to identify the specific use cases that align with your business objectives. Some common applications include:
- Customer segmentation and targeting
- Sentiment analysis and social media monitoring
- Product recommendation engines
- Churn prediction and customer retention
- Forecasting demand and optimizing supply chain
By pinpointing the areas where machine learning can add value, you can focus your efforts and resources effectively.
3. Data Collection and Preparation
Quality and quantity of data play a crucial role in the success of machine learning models. In this phase, it is imperative to gather relevant data from various sources and ensure its cleanliness and accuracy. Data preparation involves cleaning, transforming, and normalizing the data to make it suitable for analysis.
There are various tools and platforms available, such as Python libraries like Pandas and NumPy, or commercial software like Microsoft Azure or Google Cloud, which can assist in data preparation and analysis.
4. Model Selection and Training
Choosing the right machine learning algorithm is essential for achieving accurate and reliable predictions. Depending on the nature of the problem, different algorithms like linear regression, decision trees, or neural networks can be utilized.
Training the model involves feeding the algorithm with historical data and adjusting its parameters to minimize errors. This iterative process continues until the model achieves satisfactory performance.
5. Model Evaluation and Validation
After the initial training, the model needs to be evaluated and validated to ensure its reliability. This involves partitioning the data into training and testing sets to measure the accuracy and generalization ability of the model.
Cross-validation techniques like k-fold or holdout validation can be used to assess the model’s performance. It is crucial to consider factors such as precision, recall, accuracy, and F1 score during evaluation.
6. Deployment and Integration
Once the model passes validation, it can be deployed into your business operations. This involves integrating it with existing systems, whether it’s an API call to a cloud service or embedding it within your own software.
Platforms like TensorFlow, PyTorch, or scikit-learn provide libraries and tools for deploying machine learning models efficiently.
7. Monitoring and Maintenance
Machine learning models are not static entities but require continuous monitoring and maintenance. Regular checks must be performed to ensure the model’s performance, accuracy, and adaptability.
Additionally, keeping the model up-to-date with fresh data and retraining it periodically helps maintain its efficacy.
8. Overcoming Challenges
Implementing machine learning in your business may come with certain challenges, such as:
- Lack of skilled data scientists and analysts
- Data privacy and security concerns
- Obtaining and managing labeled training data
- Computational resource requirements
By being aware of these challenges, you can plan and allocate resources accordingly to overcome them.
9. FAQs
Q: Can machine learning work with small datasets?
A: Yes, machine learning can work with small datasets, but it may produce less accurate results compared to larger datasets. Techniques like data augmentation and transfer learning can help mitigate limited training data challenges.
Q: What programming languages are commonly used in machine learning?
A: Python and R are popular languages for machine learning due to their extensive libraries and frameworks that simplify the implementation of algorithms and data manipulation.
Q: How long does it take to develop and deploy a machine learning model?
A: The time required depends on various factors, including the complexity of the problem, the availability and quality of data, and the expertise of the team. Simple models can be developed and deployed within a few weeks, while more complex projects may take several months.
Conclusion
Machine learning presents a tremendous opportunity for businesses to gain a competitive edge and make data-driven decisions. By understanding the concepts, identifying relevant use cases, and following the necessary steps, you can unleash the power of AI and bring significant advancements to your organization.
References:
1. “Machine Learning: A Probabilistic Perspective” by Kevin P. Murphy
2. “Python for Data Analysis” by Wes McKinney