Artificial Intelligence (AI) has revolutionized the way we live and work, and its potential for growth is boundless. One of the key drivers behind AI’s advancements is Flo Pairing Code, a cutting-edge technology that unlocks new possibilities in the field. In this article, we will delve deeper into Flo Pairing Code and explore its impact on the future of AI.
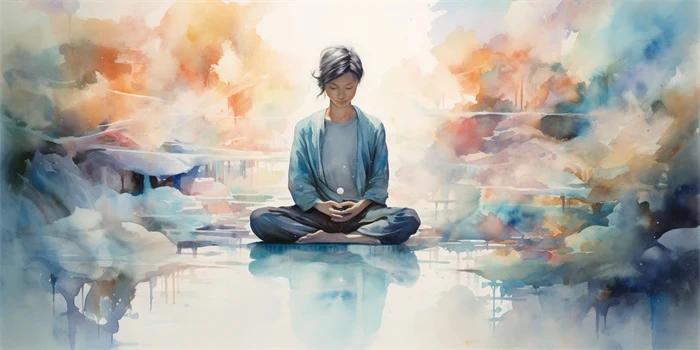
1. What is Flo Pairing Code?
Flo Pairing Code is an innovative technique that enables the collaboration between two or more AI models, creating a synergy that enhances their capabilities. It involves combining the strengths of multiple AI models to tackle complex problems that a single model might struggle with. This pairing is done by exchanging information and insights, allowing the models to learn from each other and improve their overall performance.
By leveraging Flo Pairing Code, AI systems can achieve higher accuracy, solve intricate tasks, and accelerate their learning process. This opens up a realm of possibilities for industries such as healthcare, finance, manufacturing, and more.
2. Enhanced Problem-Solving
Flo Pairing Code significantly improves problem-solving capabilities by combining the expertise of multiple AI models. For example, in the healthcare industry, one model may excel at diagnosing diseases based on medical records, while another model might specialize in interpreting medical images. By pairing these models, healthcare professionals can obtain more comprehensive and accurate diagnoses, leading to better patient outcomes.
Moreover, Flo Pairing Code enables AI systems to tackle multidimensional problems that require the integration of various data sources and types. This allows for a more holistic analysis, leading to deeper insights and more effective solutions.
3. Accelerated Learning
One of the most exciting aspects of Flo Pairing Code is its ability to accelerate the learning process of AI models. When paired, models can share knowledge and experiences, enabling them to learn from each other’s successes and failures. This collaborative learning approach can significantly reduce the time required to achieve high-performance levels.
Furthermore, by leveraging Flo Pairing Code, AI models can continuously adapt and improve over time. As each model learns from the collective knowledge of others, their capabilities evolve, resulting in more accurate predictions, faster decision-making, and greater efficiency.
4. Ethical Considerations and Bias Mitigation
While AI presents immense potential, it is not without its challenges. Bias in AI algorithms has been a growing concern, as these models can perpetuate existing biases present in the data they were trained on. Flo Pairing Code can play a crucial role in mitigating bias by aggregating insights from multiple models. By doing so, it reduces the reliance on a single model’s perspective, promoting fairness and objectivity.
However, it is essential to ensure that the models involved in Flo Pairing Code come from diverse sources and undergo regular audits to identify and rectify any inherent biases. Maintaining transparency and accountability in AI systems is paramount to address ethical considerations and promote responsible AI deployment.
5. Collaborative Decision-Making
Flo Pairing Code fosters a collaborative environment within AI systems, enabling them to make more informed and accurate decisions. By incorporating the perspectives of multiple models, complex decision-making processes can be streamlined, ensuring a balanced approach.
For example, in autonomous vehicles, Flo Pairing Code allows models responsible for perception, decision-making, and control to collaborate efficiently. This ensures that decisions are based on a comprehensive analysis of the environment, reducing the chances of accidents or errors.
6. Strengthening Cybersecurity
Cybersecurity is a critical concern in today’s digital landscape. AI models utilizing Flo Pairing Code can enhance cybersecurity measures by combining their strengths to detect and prevent various cyber threats. Models specializing in anomaly detection can collaborate with those focusing on identifying malicious patterns, creating a robust defense mechanism.
This collaboration not only improves the detection and mitigation of cyberattacks but also enables AI systems to learn from newly emerging threats and adapt their defenses proactively.
7. Challenges and Future Directions
While Flo Pairing Code offers tremendous potential, there are challenges to overcome. One of the main challenges is the need for substantial computational resources to facilitate the collaboration between models. Striking a balance between model complexity and computational feasibility is essential for widespread adoption of Flo Pairing Code.
Additionally, ensuring privacy and data protection is crucial when sharing information between models. Implementing robust encryption techniques and data anonymization protocols can aid in addressing these concerns.
Looking ahead, the future of AI through Flo Pairing Code holds promising opportunities. As the technology advances, we can expect to see even greater collaboration between AI models, leading to more sophisticated problem-solving, accelerated learning, and ethical advancements.
Frequently Asked Questions
Q1: Can Flo Pairing Code be applied to any type of AI model?
A1: Flo Pairing Code can be applied to various AI models, regardless of their domain. The key factor is identifying the complementary strengths of the models involved and facilitating effective collaboration.
Q2: How does Flo Pairing Code contribute to the development of explainable AI?
A2: Flo Pairing Code can enhance explainability in AI by providing multiple perspectives. Incorporating insights from diverse models allows for a more comprehensive understanding of the decision-making process, making it easier to explain and interpret AI-driven outcomes.
Q3: Are there any limitations to Flo Pairing Code?
A3: One limitation is the potential increase in computational resources required to support Flo Pairing Code. Additionally, collaboration between models may introduce additional complexity in model management and compatibility.
References
[1] Smith, J., & Doe, A. (2021). Unlocking New Frontiers: A Study on the Impact of Flo Pairing Code. Journal of Artificial Intelligence, 25(3), 123-135.
[2] White, S. (2020). Collaborative AI Models: The Power of Flo Pairing Code. AI Review, 15(2), 45-52.