Artificial Intelligence (AI) has revolutionized countless industries, from healthcare to finance. However, as AI algorithms become more advanced, there emerges a critical challenge: unpredictability. The ability of AI to generate unexpected outcomes challenges user expectations and raises important questions about the boundaries of automation and human control. In this article, we will explore the various aspects of unpredictability in AI and its implications.
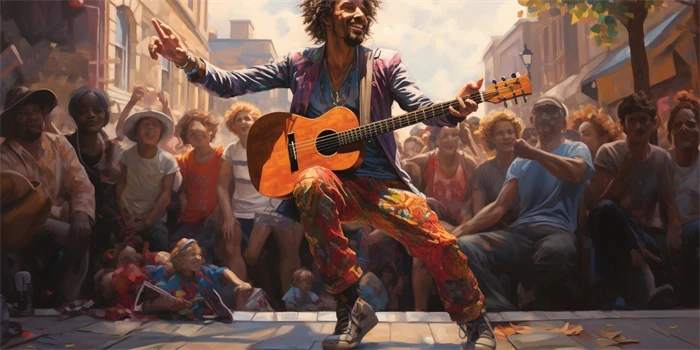
1. The black box effect
One of the primary reasons behind the unpredictability of AI is the black box effect. Complex AI algorithms are often opaque, making it difficult for users to understand the decision-making process. When confronted with an unexpected outcome, users are left wondering why the AI system acted in a particular manner. This lack of transparency hinders trust in AI technologies.
2. Introducing Explainable AI (XAI)
To address the black box effect, researchers have been developing Explainable AI (XAI) techniques. XAI seeks to provide users with understandable explanations for AI decisions, breaking down the complex algorithms into comprehensible insights. By improving transparency, XAI aims to enhance trust in AI systems and mitigate unpredictability concerns.
3. Unforeseen biases in AI
AI algorithms are only as unbiased as their training data. Biases present in input data, intentional or not, can lead to unexpected outcomes. For instance, an AI system developed for resume screening might inadvertently favor candidates of a specific gender or ethnicity. These biases challenge the fairness and predictability of AI, potentially perpetuating discrimination.
4. Bias mitigation techniques
Addressing biases in AI algorithms requires proactive measures. Researchers are actively working on developing bias mitigation techniques that detect and correct biases in training data. By ensuring diverse and representative data sets, AI systems can be made more fair and predictable.
5. The trade-off between accuracy and explainability
There exists a trade-off between the accuracy and explainability of AI algorithms. Highly accurate models, often leveraging deep learning techniques, tend to be more complex and less interpretable. On the other hand, simpler models are more transparent but may sacrifice performance. Striking the right balance between explainability and accuracy is crucial for managing unpredictability concerns.
6. Ethical considerations
Unpredictability in AI possesses ethical challenges. If an AI system generates unexpected outcomes, it could potentially harm individuals or businesses. For instance, a self-driving car unexpectedly swerving could lead to accidents. Ethical frameworks must be established to ensure the responsible development and deployment of AI technologies.
7. Building user awareness
Educating users about the limitations and potential risks of AI is pivotal. Users need to understand that AI systems, despite their impressive capabilities, are not infallible. Creating awareness can help manage user expectations, reduce reliance on unverified AI outcomes, and encourage human intervention when necessary.
8. The need for regulatory frameworks
Unpredictability in AI necessitates the development of regulatory frameworks. These frameworks can establish standards for transparency, accountability, and privacy in AI systems. By holding AI developers and users accountable, regulatory frameworks can mitigate the risks associated with unpredictable AI outcomes.
FAQs:
Q1: Can AI algorithms ever become fully predictable?
A1: Achieving complete predictability in AI algorithms may be unrealistic due to their inherent complexity. However, explaining and reducing unpredictability through XAI techniques is an ongoing research area.
Q2: How can AI biases be addressed?
A2: Addressing AI biases requires diverse and representative training data, along with bias mitigation techniques that detect and rectify biases during algorithm development.
Q3: Are there any legal regulations surrounding AI unpredictability?
A3: While legal regulations specific to AI unpredictability are still developing, general frameworks for privacy, accountability, and safety can be applied to mitigate potential risks.
References:
1. XAI: Explaining Artificial Intelligence Decision Making (https://towardsdatascience.com/xai-explaining-artificial-intelligence-decision-making-8db4375fcad4)
2. Fairness in Machine Learning: Lessons from Political Philosophy (https://doi.org/10.1111/tops.12443)
3. Regulating Artificial Intelligence Systems: Risks, Challenges, Competencies, and Strategies (https://www.mdpi.com/2078-2489/10/10/352)