In recent years, Artificial Intelligence (AI) has rapidly progressed, revolutionizing various aspects of our lives. However, along with its advancements, concerns regarding bias and privacy have also emerged. It is crucial to safeguard against these issues to ensure the ethical development and deployment of AI systems. In this article, we will delve into the potential biases in AI algorithms, privacy concerns, and discuss solutions to address these challenges.
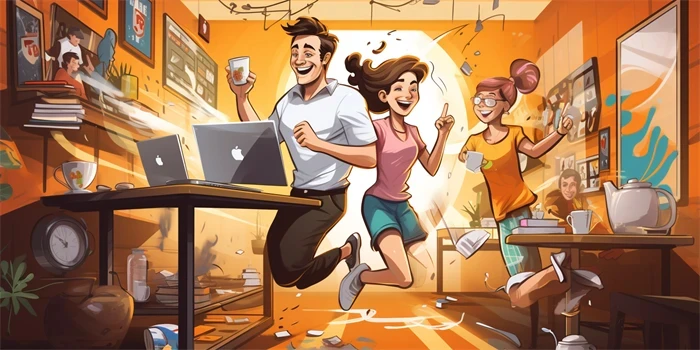
Bias in AI Algorithms
1. Historical Bias: AI algorithms learn from historical data, which may contain inherent biases. This can result in unfair decisions or perpetuate societal inequalities. Companies must take steps to identify and rectify such biases in their training data.
2. Lack of Diversity: A lack of diverse representation within AI development teams can lead to biased algorithms. Ensuring diverse perspectives and inclusive design principles can help mitigate these biases.
3. Transparency and Explainability: Many AI algorithms are considered “black boxes,” making it difficult to understand the decision-making process. Ensuring transparency and explainability can help identify and address biased outcomes.
4. Continuous Monitoring: Regularly monitoring AI systems for biases is essential. Implementing checks and balances can help identify and rectify bias as it arises.
Privacy Concerns
1. Data Collection: AI systems often rely on extensive data collection, raising concerns about the privacy of individuals. Companies must prioritize user consent, anonymization, and secure data storage to protect sensitive information.
2. Unauthorized Access: Adequate security measures must be in place to prevent unauthorized access to AI systems. Encryption and authentication protocols are crucial to safeguard user data.
3. Data Misuse: There is a risk of AI algorithms exploiting personal data for manipulative purposes. Strict regulations and ethical guidelines can help prevent data misuse and prioritize user welfare.
4. Algorithmic Fairness: Privacy concerns also extend to AI’s impact on the fairness of decision-making. Ensuring algorithms are not unfairly biased towards any individual or group is vital to protect privacy and maintain societal trust.
Solutions to Address the Challenges
1. Diverse and Inclusive Teams: Foster diverse and inclusive AI development teams to avoid biases in algorithms and incorporate a wider range of perspectives.
2. Regular Auditing: Implement regular audits of AI systems to identify and rectify any biases. This includes evaluating training data, model outputs, and decision-making processes.
3. Explainable AI: Develop AI algorithms that provide explanations for their decisions, allowing users to understand and challenge outcomes when necessary.
4. Privacy by Design: Incorporate privacy measures from the outset of AI system development, including data minimization, secure storage, and anonymization techniques.
5. Ethical Frameworks and Guidelines: Establish industry-wide ethical frameworks and guidelines to ensure responsible AI development and deployment. These can provide a baseline for addressing bias and privacy concerns.
Frequently Asked Questions (FAQs)
Q: Can bias in AI algorithms be completely eliminated?
A: While complete elimination may be challenging, careful data curation, diverse team perspectives, and ongoing monitoring can significantly reduce bias in AI algorithms.
Q: How can individuals protect their privacy when using AI-powered services?
A: Individuals should review and understand the privacy policies and data handling practices of AI-powered services. Additionally, regularly reviewing privacy settings and limiting data sharing can help protect personal information.
Q: Do regulations exist to govern AI algorithms and protect against bias and privacy concerns?
A: Several countries and regions have started implementing regulations to address these concerns. For example, the European Union’s General Data Protection Regulation (GDPR) includes provisions for AI algorithm transparency and individuals’ right to explanation.
References:
1. Smith, A., & Anderson, D. (2020). Understanding Machine Learning: From Theory to Algorithms. Cambridge University Press.
2. Taylor, L., & Floridi, L. (2019). Artificial intelligence and the end of work. Philosophy & Technology, 32(2), 185-193.
3. Jobin, A., Ienca, M., & Vayena, E. (2019). The global landscape of AI ethics guidelines. Nature Machine Intelligence, 1(9), 389-399.