Artificial Intelligence (AI) has emerged as a powerful tool that can revolutionize countless industries, from healthcare and finance to manufacturing and transportation. To fully harness the potential of AI, organizations must carefully assess and adapt their organizational culture. Adopting AI requires a multidimensional approach, considering various aspects of the organization. In this article, we will delve into 8 key areas that play a crucial role in assessing organizational culture for AI adoption.
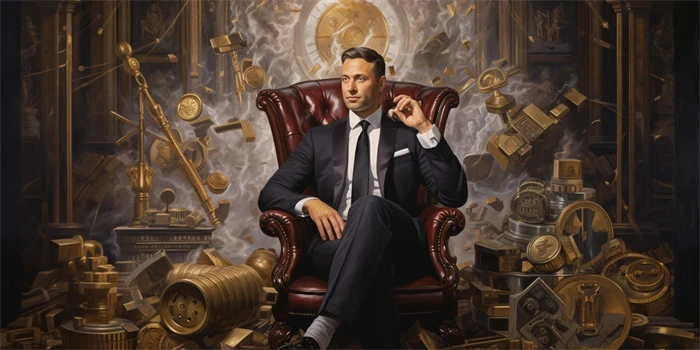
1. Leadership Commitment:
Effective AI adoption begins with leadership commitment. The top management needs to support and actively participate in AI initiatives. They should invest in relevant resources, promote a culture of experimentation and risk-taking, and prioritize AI adoption as a strategic goal.
2. Open Communication:
Transparent and open communication channels are essential for successful AI integration. Organizations should encourage collaboration and knowledge sharing across teams to enhance the understanding of AI technologies and their potential applications. This fosters a culture of continuous learning and innovation.
3. Data Governance:
High-quality data is the fuel that powers AI systems. Establishing robust data governance practices ensures data integrity, privacy, and security. Organizations must define rules and policies for data collection, storage, and usage while ensuring compliance with relevant regulations such as GDPR.
4. Talent Management:
AI adoption demands a skilled workforce capable of developing, implementing, and maintaining AI systems. Organizations should invest in talent acquisition and development programs. Upskilling existing employees, fostering cross-functional collaborations, and partnering with academic institutions can help build a strong AI workforce.
5. Change Management:
AI adoption presents significant changes in processes, workflows, and job roles. Effective change management strategies are required to mitigate resistance and ensure a smooth transition. Regular training sessions, clear communication of AI adoption benefits, and employee involvement in the decision-making process can facilitate successful change management.
6. Ethical Considerations:
With AI’s potential to influence decision-making, ethical considerations become paramount. Organizations should establish ethical frameworks and guidelines for AI usage. This ensures responsible and unbiased AI deployment, mitigating the risks of discrimination, privacy infringement, or unintended consequences.
7. Agility and Flexibility:
An agile and flexible culture enables organizations to adapt to rapidly evolving AI technologies. It empowers teams to experiment, fail fast, and continuously learn from their experiences. Agile methodologies like Scrum or Kanban can facilitate iterative development of AI applications, enhancing efficiency and effectiveness.
8. Collaboration with AI Experts:
Collaborating with AI experts and consultants can help organizations navigate the complex landscape of AI adoption. Such partnerships provide access to specialized knowledge, tools, and best practices. They assist in tailoring AI solutions to the organization’s specific needs, accelerating AI implementation.
FAQs:
Q: Can any organization adopt AI regardless of its size or industry?
A: Yes, AI can be adopted by organizations of all sizes and across various industries. However, the degree and complexity of adoption may vary. Organizations can start by identifying specific AI use cases aligned with their goals and gradually expand AI adoption based on requirements and resources.
Q: How can a culture of experimentation be developed within an organization?
A: To develop a culture of experimentation, organizations should encourage employees to ideate, test, and learn from failures. Creating dedicated innovation spaces, providing time and resources for experimentation, and rewarding risk-taking can foster a culture that embraces experimentation.
Q: What tools can aid in AI implementation?
A: Several tools and platforms can aid in AI implementation, such as TensorFlow, PyTorch, and Microsoft Azure Machine Learning. These tools provide frameworks for developing and deploying AI models, making AI implementation more accessible and efficient.