With the rapid growth in data generation and the increasing complexity of data analysis, organizations are turning to artificial intelligence (AI) technology to enhance the efficiency and accuracy of their data analysis processes. AI technology provides advanced algorithms and computational power that can handle large volumes of data and extract valuable insights. In this article, we will explore how AI technology improves data analysis from various perspectives.
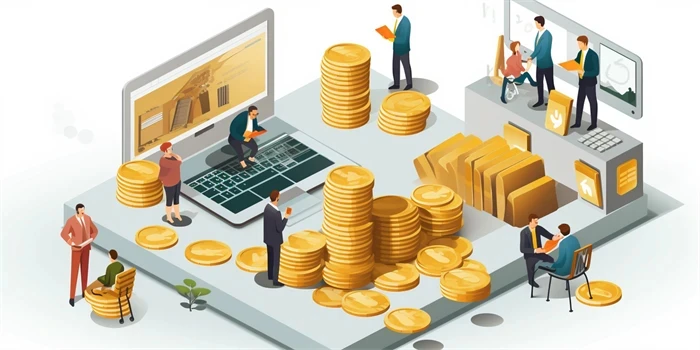
1. Automated Data Cleaning and Preprocessing
AI technology enables automated data cleaning and preprocessing, reducing the time and effort required in these crucial steps of data analysis. By leveraging machine learning algorithms, AI systems can identify and correct errors, handle missing values, and transform raw data into a structured format suitable for analysis.
2. Advanced Predictive Analytics
AI-powered predictive analytics algorithms can analyze historical data patterns and make accurate predictions about future events or trends. This enables organizations to proactively identify opportunities, anticipate customer behavior, and make data-driven decisions.
3. Natural Language Processing (NLP)
NLP is a branch of AI that focuses on the interaction between computers and human language. AI-powered NLP tools can analyze unstructured text data, such as customer reviews or social media posts, to extract sentiment, identify topics, or categorize content. This capability enhances the analysis of textual data, providing valuable insights for decision-making.
4. Image and Video Analysis
AI technology can also analyze images and videos, extracting relevant information and patterns. For example, in the healthcare industry, AI algorithms can analyze medical images to detect diseases or anomalies, supporting accurate diagnosis and treatment planning.
5. Real-time Data Analysis
AI technology enables real-time data analysis, allowing organizations to process and analyze data streams as they are generated. This is especially valuable in industries such as finance, where real-time insights are critical for making timely investment decisions.
6. Automated Anomaly Detection
AI-powered anomaly detection algorithms can automatically identify unusual patterns or outliers in data, flagging potential fraud or anomalies that require further investigation. This helps organizations detect anomalies more efficiently, improving their ability to prevent and mitigate risks.
7. Collaborative Filtering
Collaborative filtering is a technology widely used in recommendation systems. By analyzing user behavior and preferences, AI algorithms can recommend personalized products or content to individuals, enhancing customer satisfaction and driving business revenue.
8. Machine Learning Model Optimization
AI technology provides advanced optimization algorithms that can fine-tune machine learning models, improving their performance and accuracy. These algorithms automatically adjust model parameters and hyperparameters, saving data scientists significant time and effort in manual optimization.
9. Data Visualization
AI-powered data visualization tools can transform complex data into visual representations, making it easier for analysts and decision-makers to understand and interpret the insights. These tools support interactive dashboards, charts, and graphs, enhancing the communication of data analysis results.
10. Automated Report Generation
AI technology can automatically generate reports summarizing the key findings and insights from data analysis. By eliminating the manual effort in report writing, organizations can save time and ensure consistent reporting standards across different projects or departments.
11. Enhanced Cybersecurity
AI algorithms can analyze vast amounts of data in real-time to detect and prevent cybersecurity threats. They can identify patterns indicative of malicious activities, helping organizations proactively protect their data and systems from cyber attacks.
12. AI for Data Quality Assessment
AI technology can assess the quality of data by analyzing factors such as completeness, accuracy, consistency, and validity. By automatically evaluating the data quality, organizations can identify and address data issues, ensuring the reliability and credibility of their analysis results.
13. Integration of Multiple Data Sources
AI technology enables the integration of data from multiple sources, such as databases, APIs, or external websites. This integrated data can provide a comprehensive view of the business or industry, enabling more accurate and insightful analysis.
14. AI-Powered Chatbots for Data Analysis Queries
AI-powered chatbots can assist users in querying and retrieving data analysis results. They can understand natural language queries and provide relevant answers or visualizations, making it easier for non-technical users to access and understand analytical insights.
15. Explainability and Trust in AI
One of the critical challenges in AI-powered data analysis is the lack of transparency and interpretability of AI algorithms. However, research is being conducted to develop explainable AI methods that can provide insights into how AI models make predictions or decisions. This enhances trust in AI and allows users to understand and validate the analysis results.
Frequently Asked Questions
1. Can AI completely replace human data analysts?
No, AI technology augments human data analysts by automating repetitive tasks, enhancing efficiency, and providing insights from large datasets. Human analysts bring domain expertise, critical thinking, and the ability to interpret complex results?aspects that AI currently lacks.
2. Are AI-powered data analysis tools expensive?
The cost of AI-powered data analysis tools can vary depending on the complexity and capabilities of the software. However, with advancements in AI technology and increased competition, more affordable options are becoming available, particularly for smaller businesses or individual users.
3. What are the ethical considerations in AI-powered data analysis?
AI-powered data analysis raises concerns about privacy, bias, and the potential for unintended consequences. It is crucial for organizations to implement ethical frameworks and ensure that AI technologies are developed and deployed responsibly to mitigate such risks.
References
[1] Miller, S. (2020). AI in Data Analysis: Pros and Cons. Retrieved from https://almabetter.com/blog/ai-in-data-analysis-pros-and-cons
[2] Kumaravel, K. (2021). AI Applications in Data Analysis and Insights. Retrieved from https://www.analyticsinsight.net/ai-applications-in-data-analysis-and-insights/