Data analysis and interpretation play a crucial role in various fields, including business, healthcare, and research. With the advancements in artificial intelligence (AI), there has been a significant impact on improving the accuracy and efficiency of these processes. In this article, we will explore how AI can enhance data analysis and interpretation from multiple perspectives.
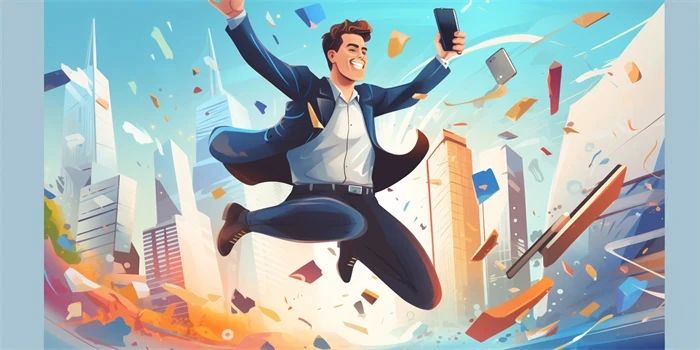
1. Automated Data Cleaning and Pre-processing
Data analysis often involves working with large datasets that can be messy and contain errors. AI algorithms can automate the cleaning and pre-processing of data, removing inconsistencies, duplicates, and missing values. This automation not only improves efficiency but also ensures the accuracy of subsequent analyses.
Additionally, AI algorithms can transform raw data into a standardized format, making it easier to analyze and interpret the information. Such automation saves valuable time and allows analysts to focus more on the actual analysis rather than spending hours on data preparation.
2. Intelligent Data Visualization
Understanding complex datasets can be challenging, especially when dealing with numerous variables and correlations. AI-powered data visualization tools provide interactive graphical representations that enhance data interpretation. By using algorithms to identify patterns and relationships, these tools can create visualizations that highlight key insights and trends within the data.
One popular tool is Tableau, which allows users to create interactive dashboards and visualizations from various data sources. These visualizations enable analysts to quickly grasp complex information, leading to more accurate interpretations.
3. Efficient Pattern Recognition
AI algorithms excel in pattern recognition, which is beneficial for data analysis. Through machine learning techniques, AI systems can identify hidden patterns and anomalies within datasets. This capability enables analysts to make accurate predictions and identify potential outliers or errors that could influence the analysis results.
For example, in fraud detection, AI-based systems can identify unusual transaction patterns and flag them for further investigation. By automating this process, the accuracy and efficiency of detecting fraudulent activities are significantly improved.
4. Natural Language Processing (NLP)
Interpreting textual data, such as customer reviews or research papers, can be time-consuming and challenging. AI-powered NLP techniques can extract relevant information, sentiment, and topics from unstructured textual data.
Tools like Google’s Cloud Natural Language API or IBM Watson’s NLP services can analyze large volumes of text, classify sentiments, extract entities, and detect themes. These capabilities enhance the efficiency of data analysis by automating the extraction of valuable insights from textual data.
5. Predictive Analytics
AI algorithms significantly enhance predictive analytics by using historical data to forecast future outcomes. By capturing complex relationships and patterns within the data, these algorithms can generate more accurate predictions.
In fields such as healthcare, predictive analytics can be used to predict disease progression or patient outcomes based on various factors. This enables healthcare professionals to make informed decisions and provide personalized treatments.
6. Efficient Resource Allocation
Data analysis often requires substantial computational resources. AI algorithms can optimize resource allocation by automating tasks and efficiently distributing computing power. This enables analysts to process and interpret large datasets in less time and with lower costs.
7. Contextual Data Integration
Data analysis frequently involves integrating multiple data sources to gain a comprehensive understanding. AI algorithms enable the integration of diverse datasets by contextualizing the information and providing a more holistic view.
For instance, in marketing, AI-powered systems can integrate data from customer demographic information, purchasing history, and online behavior to provide targeted recommendations and personalized advertisements.
8. Real-time Data Analysis
A key advantage of AI-powered data analysis is the capability to process and interpret data in real-time. Traditional methods often struggle with the volume and speed at which data is generated, limiting the ability to make timely decisions.
AI algorithms, such as Apache Kafka, enable real-time data streaming and analysis. This allows businesses to respond promptly to changing trends and make data-driven decisions almost instantly.
9. Improved Data Security
Data security is a concern when handling sensitive information. AI systems can enhance data security by detecting and preventing unauthorized access or data breaches.
Machine learning algorithms can identify unusual user behavior, flag potential security threats, and protect sensitive data from unauthorized access. This strengthens data analysis by ensuring the integrity and confidentiality of the information.
Conclusion
Artificial intelligence has revolutionized data analysis and interpretation, making them more accurate and efficient. Through automated data cleaning, intelligent visualization, pattern recognition, natural language processing, predictive analytics, resource allocation optimization, contextual data integration, real-time analysis, and improved data security, AI algorithms have become indispensable tools in various industries. As AI technology continues to advance, we can expect even greater improvements in the accuracy, efficiency, and depth of data analysis and interpretation.
Frequently Asked Questions (FAQs)
Q1: Can AI completely replace human analysts in data analysis?
A1: No, AI cannot entirely replace human analysts. While AI algorithms can automate parts of the data analysis process, human expertise is still crucial for interpreting results, providing context, and making informed decisions based on the analysis.
Q2: How can AI help in anomaly detection?
A2: AI algorithms can identify anomalies by learning patterns from historical data. By comparing observed data against these patterns, AI systems can flag potential outliers or anomalies for further investigation.
Q3: What are the limitations of AI-powered data analysis?
A3: AI-powered data analysis may face challenges with biased data, limited interpretability of black-box models, and potential ethical concerns related to data privacy and fairness.
References:
1. AltexSoft. (2021). How Harnessing Data Mining in Healthcare Enhances Diagnostics and Treatment. Retrieved from https://www.altexsoft.com/blog/datascience/how-data-mining-in-healthcare-enables-diagnostics-and-treatment-enhancement/
2. Tableau. (n.d.). Tableau Overview. Retrieved from https://www.tableau.com/