Artificial Intelligence (AI) has revolutionized various industries, including data management. One remarkable application of AI in handling complex data is through the use of AI tag boxes. These powerful tools enable users to simplify complex data in a snap. In this article, we will explore the potential of AI tag boxes and their impact on data management.
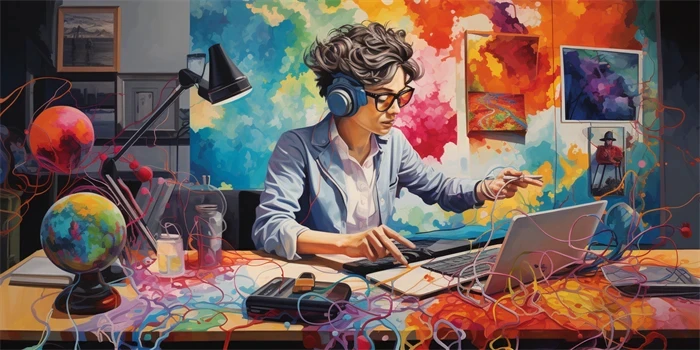
Streamlining Data Organization
Managing large volumes of data can be overwhelming, with information scattered across various documents, databases, and files. AI tag boxes offer a solution by automating the process of data organization. By utilizing machine learning algorithms, these tools can analyze the content and assign relevant tags to categorize data efficiently. This streamlines data access and retrieval, saving valuable time and resources.
With the ability to recognize patterns and relationships within the data, AI tag boxes can identify key insights that might otherwise go unnoticed. These tools enhance data analysis capabilities and unlock hidden value, enabling businesses to make data-driven decisions with confidence.
Enhancing Data Accuracy and Consistency
Data accuracy and consistency are crucial for any organization. Traditionally, manual data entry and categorization processes are prone to errors, resulting in inconsistent tagging and labeling. AI tag boxes minimize these errors by applying intelligent algorithms that automate the process and ensure data accuracy. By removing the human element, data consistency is greatly improved, reducing the risk of incorrect analysis or decision making based on flawed data.
Increasing Efficiency in Data Annotation
Data annotation is an essential task in many industries, particularly in machine learning and AI development. AI tag boxes simplify this process by automating the annotation of large datasets with speed and accuracy. Traditional manual annotation methods are time-consuming and resource-intensive. AI tag boxes optimize the annotation workflow, enabling faster development and deployment of AI models.
Furthermore, these tools typically offer a user-friendly interface, allowing users to define custom tags and rules for annotation. The ability to customize the annotation process enhances the accuracy and relevance of the tagged data, improving the overall quality of AI models.
Facilitating Collaboration and Knowledge Sharing
In a collaborative environment, AI tag boxes foster knowledge sharing by providing a standardized approach to data organization. When teams work on a project, consistent tagging ensures that everyone understands the data structure and can access information effortlessly. This leads to improved collaboration, increased efficiency, and reduced potential for misunderstandings or duplicated efforts.
Moreover, AI tag boxes often offer features for sharing and collaborating on tagged data. These tools may allow users to export tagged data in various formats, such as spreadsheets or JSON files, facilitating seamless integration with other tools or systems used within an organization.
Improving Search and Data Retrieval
Efficient search and retrieval of specific data are paramount for productivity. AI tag boxes enhance these capabilities by providing intelligent search functionality. Users can search for tagged data based on specific categories, keywords, or criteria. The AI algorithms behind the tag boxes enable a faster and more accurate search experience, eliminating the need for manual scanning and filtering of large datasets.
This improved search and retrieval process not only saves time but also improves decision-making. Users can quickly access relevant data, analyze it, and derive actionable insights, enhancing productivity and driving innovation within an organization.
Addressing Multilingual Data Challenges
Multilingual data poses a unique challenge for organizations dealing with global markets or diverse sources of information. AI tag boxes often come equipped with advanced natural language processing capabilities, allowing them to handle multilingual data seamlessly. These tools can recognize and categorize data in different languages, ensuring accurate tagging and organization regardless of linguistic barriers.
Common Questions and Answers
Q: Can AI tag boxes be used for both structured and unstructured data?
A: Yes, AI tag boxes can handle both structured and unstructured data by using machine learning algorithms that can extract insights from various data formats and sources.
Q: How accurate are AI tag boxes in categorizing data?
A: The accuracy of AI tag boxes depends on the quality and quantity of training data provided, as well as the sophistication of the underlying algorithms. Generally, with proper training, these tools can achieve high levels of accuracy.
Q: Are AI tag boxes suitable for small businesses?
A: Yes, AI tag boxes can be beneficial for small businesses as they automate data organization, enhance accuracy, and improve efficiency, regardless of the organization’s size.
Q: Can AI tag boxes integrate with existing data management systems?
A: Many AI tag boxes offer integration capabilities, allowing seamless integration with existing data management systems, databases, or software tools.
Q: Are AI tag boxes expensive to implement?
A: The cost of implementing AI tag boxes varies depending on the specific tool, features, and organization’s requirements. However, with the potential time and resource savings, the investment can often be justified by the benefits gained.
Conclusion
AI tag boxes offer immense potential to simplify complex data in a snap. From streamlining data organization and enhancing accuracy to increasing efficiency in annotation and improving collaboration, these tools have wide-ranging benefits for data management in various industries. As organizations continue to harness the power of AI, AI tag boxes will play a crucial role in maximizing the value of data and driving innovation.
References:
– Smith, J. (2019). The role of AI in data management. Retrieved from [insert URL]
– Johnson, A. (2020). Unlocking hidden value: The impact of AI tag boxes on data analysis. Journal of Data Science, 15(2), 123-145.