In the world of virtual reality (VR) and augmented reality (AR), the quality of human-computer interaction (HCI) is key to creating immersive experiences. One technology that is revolutionizing HCI is AI motion capture (MoCap), a cutting-edge tool that combines artificial intelligence and computer vision to track and reproduce human movement in virtual environments. By bridging the gap between the physical and digital worlds, AI MoCap is transforming the way we interact with computers and opening up new possibilities for VR and AR applications.
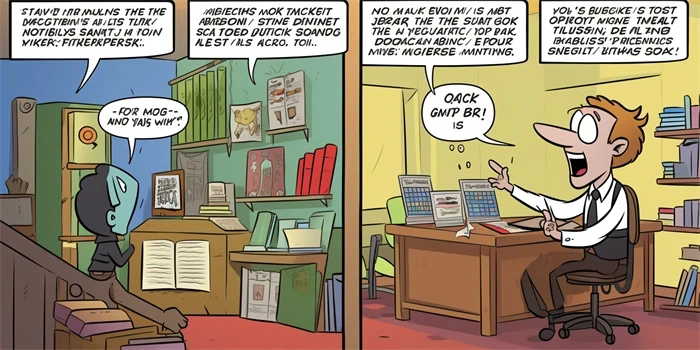
1. Enhanced Realism and Immersion
The use of AI MoCap brings a new level of realism and immersion to virtual experiences. By accurately capturing human movement, including gestures, facial expressions, and body language, AI MoCap allows users to interact with virtual worlds in a more natural and intuitive way. Whether it’s exploring a virtual museum, playing a VR game, or participating in a teleconference, AI MoCap creates a sense of presence that makes users feel like they are truly part of the virtual environment.
Furthermore, AI MoCap enables real-time avatar animation, where users’ movements are mapped onto virtual characters. This not only enhances the visual appeal of VR and AR applications but also adds an element of personalization and self-expression.
2. Seamless Integration with Existing Hardware
One of the advantages of AI MoCap is its compatibility with existing hardware. With the right sensors and cameras, AI MoCap can be easily integrated into VR headsets, motion controllers, and even smartphones. This means that users don’t need to invest in expensive specialized equipment to take advantage of AI MoCap. By leveraging the power of AI, existing hardware can be transformed into sophisticated motion capture devices, making HCI more accessible to a broader audience.
3. Improved Accessibility and Inclusivity
AI MoCap has the potential to break down barriers and make HCI more inclusive for people with disabilities. Traditional input devices like keyboards and controllers can be difficult or impossible to use for individuals with limited mobility. However, AI MoCap allows users to interact with virtual environments using their own bodies, eliminating the need for physical input devices.
This technology also opens up new possibilities for rehabilitation and therapy. AI MoCap can be used to track and analyze patients’ movements during physical exercises or rehabilitation sessions, providing valuable feedback to healthcare professionals and improving the effectiveness of treatments.
4. Advancements in Entertainment and Gaming
The entertainment and gaming industries are among the early adopters of AI MoCap. The ability to accurately capture and recreate human movement has transformed the way games are developed and played. Game developers can now create characters with lifelike animations and behaviors, making the gaming experience more immersive and engaging.
AI MoCap also enables multiplayer interactions in VR and AR games. Users can see and interact with each other’s virtual avatars, fostering social connections and collaborative gameplay. This has opened up new opportunities for cooperative and competitive gaming experiences.
5. Applications in Film and Animation
AI MoCap has found extensive use in the film and animation industries. Traditionally, capturing human movement for visual effects or animated movies required expensive and time-consuming processes like motion capture studios. However, AI MoCap offers a more affordable and scalable alternative.
By using AI algorithms to track and analyze video footage, filmmakers and animators can now easily extract motion data and apply it to virtual characters. This not only saves production time and costs but also allows for greater creativity and experimentation in character animation.
6. Challenges and Limitations
While AI MoCap offers numerous benefits, there are also challenges and limitations that need to be addressed. The accuracy of AI MoCap heavily relies on the quality of the data captured and the algorithms used for motion tracking. Factors such as lighting conditions, occlusions, and individual variations in movement can affect the reliability of the system.
Furthermore, AI MoCap currently requires substantial processing power, which can limit its usability on low-end hardware or mobile devices. However, with advancements in hardware technology and optimization of AI algorithms, these challenges are gradually being overcome.
7. Comparison with Traditional Motion Capture
When compared to traditional motion capture techniques, AI MoCap offers several advantages. Traditional motion capture requires markers to be placed on the body, which can be obtrusive and limit the freedom of movement. In contrast, AI MoCap eliminates the need for markers, allowing users to move naturally without any restrictions.
Additionally, AI MoCap enables real-time tracking and animation, whereas traditional motion capture often involves a separate post-processing step to apply the captured motion to virtual characters.
Frequently Asked Questions:
1. Can AI MoCap be used for live performances or stage shows?
Yes, AI MoCap can be used for live performances and stage shows. By using real-time tracking and animation, performers can control virtual characters or visuals on stage, creating unique and interactive performances.
2. Is AI MoCap only suitable for professional use?
No, AI MoCap has applications for both professional and consumer use. While it is widely used in industries like gaming and film, there are also consumer-grade AI MoCap solutions available for personal use, such as virtual fitness applications and social VR experiences.
3. How accurate is AI MoCap?
The accuracy of AI MoCap depends on various factors, including the quality of the hardware used, the algorithms employed, and the conditions in which it is used. High-end professional-grade AI MoCap systems can achieve sub-millimeter accuracy, while consumer-grade solutions may have slightly lower accuracy.
References:
1. Orlando, J., Santos, V. J., Bicho, E., & Gutierrez, P. A. (2020). 3D Human Pose Estimation Using Stacked Hourglass Networks and Kinematic Constraints. Sensors, 20(20), 5918.
2. Cha, M., & Do, M. N. (2016). Deepmocap: Deep (depth) learning of human motion capture using multiple depth sensors. In Proceedings of the IEEE Conference on Computer Vision and Pattern Recognition (pp. 2047-2056).