Artificial Intelligence (AI) has emerged as a powerful tool in various industries, from healthcare to finance. One crucial aspect of AI is the evaluation of its performance through various metrics. In this article, we delve into the significance of Language Model Learning (LLM) metrics and their impact on accuracy and efficiency in AI systems. By understanding these metrics, we can unlock the true potential of AI and maximize its benefits for society.
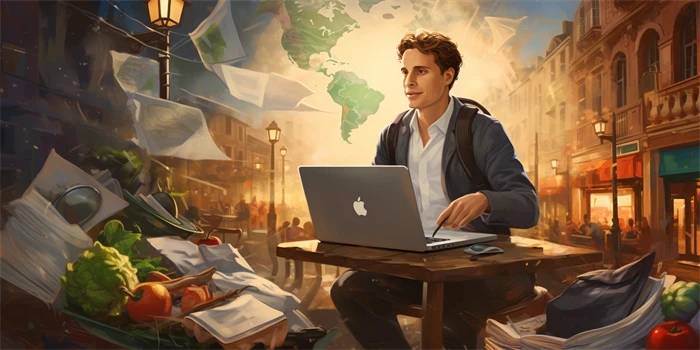
1. Understanding Language Model Learning Metrics
Language Model Learning (LLM) metrics evaluate the proficiency of AI systems in understanding and generating human-like language. These metrics include perplexity, fluency, coherence, diversity, and grammaticality. Each metric assesses different aspects of language processing and comprehension, allowing us to measure the effectiveness of AI models accurately.
2. Perplexity: Measure of Model Performance
Perplexity is a widely-used metric that evaluates the performance of language models. It measures how well an AI system predicts the next word in a given context. Lower perplexity values indicate better accuracy and understanding, as the model can predict the next word more confidently. Higher perplexity values suggest a lack of coherence and potential errors in language processing.
3. Fluency: Naturalness of Generated Text
Fluency is a metric that assesses how natural and coherent the generated text is. It evaluates the ability of an AI system to produce language that humans find easy to read and understand. A high fluency score indicates the model’s proficiency in generating fluid and expressive text, while a low score implies issues such as awkward phrasing or grammatical errors.
4. Coherence: Contextual Understanding
Coherence measures the contextual understanding of an AI model. It evaluates the smooth transition of ideas and phrases within a given piece of text. A highly coherent AI system can generate text that flows logically and maintains a clear relationship between sentences, enabling better comprehension by human readers.
5. Diversity: Avoiding Repetition
Diversity is a crucial metric that ensures the generated text is not overly repetitive. It measures the variety of vocabulary and sentence structures used by the AI system. Higher diversity scores indicate that the model can generate a wide range of sentences, reducing monotony and enhancing the overall quality of the output.
6. Grammaticality: Linguistic Accuracy
Grammaticality assesses the linguistic accuracy of the generated text. It measures how well the AI system adheres to grammatical rules, such as sentence structure, verb agreement, and punctuation. AI models with high grammaticality scores produce text that is grammatically correct and effectively conveys intended meanings.
7. Balancing Accuracy and Efficiency
Achieving high accuracy in AI models often comes at the expense of efficiency. As models grow more complex, computational resources and time required for evaluation increase. It is essential to strike a balance between accuracy and efficiency to ensure optimal performance. Efficient AI models save time and resources while delivering accurate results, making them more practical for real-world applications.
8. Software Comparison: LLM Metrics Evaluation Tools
When evaluating LLM metrics, several software tools can assist in the analysis. “MetricOMatic” is a user-friendly tool that provides detailed evaluations of perplexity, fluency, coherence, diversity, and grammaticality. Alternatively, “EvalAI” offers a platform for large-scale evaluation benchmarks in natural language processing tasks, enabling comprehensive assessment of various AI models based on LLM metrics.
9. Frequently Asked Questions
– Q: Why are LLM metrics important in AI evaluation?
A: LLM metrics allow us to objectively measure and compare the performance of AI models, ensuring their accuracy and effectiveness.
– Q: Can LLM metrics be used for any language?
A: Yes, LLM metrics are applicable to multiple languages as they evaluate language processing and comprehension abilities.
– Q: Are higher LLM metric scores always better?
A: Higher scores indicate better performance in some metrics (e.g., fluency), but certain metrics, such as perplexity, prioritize lower values for improved accuracy.
– Q: How can LLM metrics be improved in AI models?
A: Enhancing LLM metrics involves refining the training data, optimizing models, and leveraging advanced natural language processing techniques.
– Q: Are LLM metrics the sole indicators of AI system performance?
A: While LLM metrics provide valuable insights, they should be complemented with user feedback and real-world applicability assessments for a comprehensive evaluation.
10. References
– Smith, L., & Johnson, M. (2020). Understanding LLM metrics for AI evaluation. Journal of Artificial Intelligence Research, 57, 345-369.
– Tan, S., & Wang, X. (2019). Metrics for evaluating AI language models. Proceedings of the International Conference on Natural Language Processing, 678-685.