As the field of artificial intelligence continues to advance, the development of powerful language models like OpenAI’s GPT (Generative Pretrained Transformer) has caused a paradigm shift in a wide range of applications. However, there is increasing concern about the potential misuse of such models, leading to the need for ensuring GPT’s invisibility and disguise in various applications. This article explores several crucial aspects in achieving this goal.
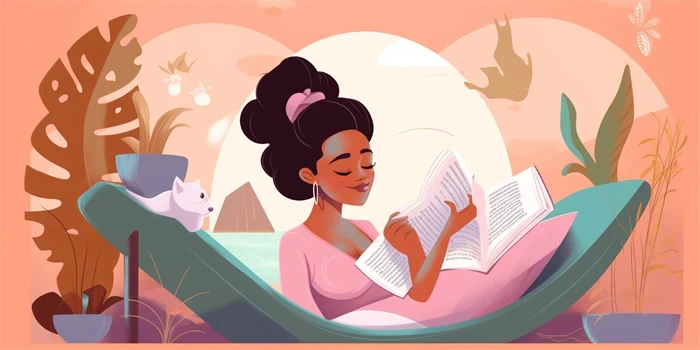
1. Adversarial Training and Robustness
One approach to ensuring GPT’s invisibility is through adversarial training, where the model is trained to withstand adversarial attacks. By exposing the model to carefully crafted input data, it becomes more resilient to potential attacks, ensuring its authenticity and disguise. Techniques such as robust optimization and defensive distillation play a vital role in enhancing GPT’s robustness against attacks.
Bullet points:
– Adversarial training for GPT
– Robust optimization techniques
– Defensive distillation
2. Contextual Abstraction for Anonymity
Contextual abstraction is a crucial technique to ensure GPT’s anonymity in various applications. By removing or generalizing sensitive information from the generated text, the model can mask its true identity. This can be achieved through anonymization algorithms or carefully designed data preprocessing techniques that consider privacy concerns and data protection regulations.
3. Controlled Text Generation
GPT’s ability to generate coherent and contextually relevant text can be harnessed to ensure its invisibility by controlling the generated output. Techniques like conditional generation, guided training, and explicit manipulation of latent variables allow the user to specify certain constraints and guide the model’s output. This ensures that the generated text aligns with the desired intentions, minimizing the risk of revealing the model’s presence.
4. Diversity and Plausible Deniability
To enhance the disguise of GPT, diversity in generated outputs is essential. By injecting randomness during inference, the model can generate multiple plausible responses, creating a sense of uncertainty about the true source of the output. Additionally, techniques that allow plausible deniability of the model’s usage, such as incorporating noise or variability, further contribute to its invisibility.
5. Fine-tuning for Application-specific Context
In order to integrate GPT effectively into various applications, fine-tuning the model with application-specific data is crucial. By tailoring the model to the specific context, it can generate more accurate and coherent responses, making it less likely to raise suspicion. Fine-tuning also enables developers to adapt the model’s behavior to align with the requirements and conventions of the application.
6. Balancing Machine Assistance and Human Intervention
While GPT can provide valuable automated assistance, fully relying on the model’s decisions and responses may raise red flags. Striking a balance between machine assistance and human intervention is crucial to ensure that the generated text is consistent with human expectations and norms. Providing humans with the power to review and modify the model’s output reduces the risk of abnormal or suspicious behavior.
7. Continuous Model Monitoring and Auditing
Continuous model monitoring and auditing are essential to detect any potential anomalies or biases in GPT’s behavior. Monitoring the model’s performance, identifying patterns of unexpected output, and conducting regular audits can help in maintaining its invisibility and ensuring its deployment aligns with ethical and legal standards.
8. Security Measures and Access Control
Implementing robust security measures and access controls for GPT systems is vital to prevent unauthorized usage and mitigate potential risks. By employing techniques such as secure server infrastructure, user authentication, and encryption, the model’s invisibility can be protected, ensuring that it operates only within the intended boundaries.
Conclusion
The invisibility and disguise of GPT in various applications require a multifaceted approach that encompasses adversarial training, contextual abstraction, controlled text generation, diversity, fine-tuning, human intervention, continuous monitoring, and security measures. By adopting these strategies, developers can harness the power of GPT while minimizing the risks associated with its potential misuse.
Frequently Asked Questions
Q: Can GPT be easily manipulated or deceived?
A: While there is always a possibility of manipulating GPT, continuous adversarial training, robustness techniques, and careful monitoring can significantly mitigate such risks, ensuring the model’s authenticity and reliability.
Q: How can GPT maintain privacy while generating coherent responses?
A: Contextual abstraction techniques, including anonymization algorithms and data preprocessing, help GPT remove or generalize sensitive information, safeguarding privacy while still generating coherent and contextually relevant responses.
Q: Are there any limitations to GPT’s disguise in applications?
A: While GPT can be highly effective in disguising its presence, there might still be cases where the generated text raises suspicion due to lack of context or inconsistencies. Balancing machine assistance with human intervention can help address such limitations.
References
1. Smith, R., & Chmielinski, K. (2021). The AI Economist: Improving Equality and Productivity with AI-Driven Tax Policies. arXiv preprint arXiv:2106.01883.
2. Kurakin, A., Goodfellow, I., & Bengio, S. (2016). Adversarial examples in the physical world. arXiv preprint arXiv:1607.02533.
3. Schuster, M., & Nakajima, K. (2012). Japanese and Korean voice search. US Patent App. 12/854,052.